Screening patients to find suitable participants for clinical trials is a labor-intensive, expensive, and error-prone task but AI could soon fix that.
A team of researchers from Brigham and Women’s Hospital, Harvard Medical School, and Mass General Brigham Personalized Medicine, conducted a study to see if an AI model could process medical records to find suitable clinical trial candidates.
They used GPT-4V, OpenAI’s LLM with image processing, enabled by Retrieval-Augmented Generation (RAG) to process potential candidates’ electronic health records (EHR) and clinical notes.
LLMs are pre-trained using a fixed dataset and can only answer questions based on that data. RAG is a technique that enables an LLM to retrieve data from external data sources like the internet or an organization’s internal documents.
When participants are selected for a clinical trial, their suitability is determined by a list of inclusion and exclusion criteria. This normally involves trained staff combing through EHRs of hundreds or thousands of patients to find those that match the criteria.
The researchers collected data from a trial that aimed to recruit patients with symptomatic heart failure. They used that data to see if GPT-4V with RAG could do the job more efficiently than study staff did while maintaining accuracy.
The structured data in the EHRs of potential candidates could be used to determine 5 out of 6 inclusion and 5 out of 17 exclusion criteria for the clinical trial. That’s the easy part.
The remaining 13 criteria needed to be determined by interrogating unstructured data in each patient’s clinical notes, which is the labor-intensive part the researchers hoped AI could assist with.
🔍Can @Microsoft @Azure @OpenAI’s #GPT4 perform better than a human for clinical trial screening? We asked that question in our most recent study and I am extremely excited to share our results in preprint:https://t.co/lhOPKCcudP
Integrating GPT4 into clinical trials is not…— Ozan Unlu (@OzanUnluMD) February 9, 2024
Results
The researchers first obtained structured assessments completed by study staff and clinical notes for the past two years.
They developed a workflow for a clinical note-based question-answering system powered by RAG architecture and GPT-4V and called this workflow RECTIFIER (RAG-Enabled Clinical Trial Infrastructure for Inclusion Exclusion Review).
Notes from 100 patients were used as a development dataset, 282 patients as a validation dataset, and 1894 patients as a test set.
An expert clinician completed a blinded review of patients’ charts to answer the eligibility questions and determine the “gold standard” answers. These were then compared to the answers from the study staff and RECTIFIER based on the following criteria:
- Sensitivity – The ability of a test to correctly identify patients who are eligible for the trial (true positives).
- Specificity – The ability of a test to correctly identify patients who are not eligible for the trial (true negatives).
- Accuracy – The overall proportion of correct classifications (both true positives and true negatives).
- Matthews correlation coefficient (MCC) – A metric used to measure how good the model was at selecting or excluding a person. A value of 0 is the same as a coin flip and 1 represents getting it right 100% of the time.
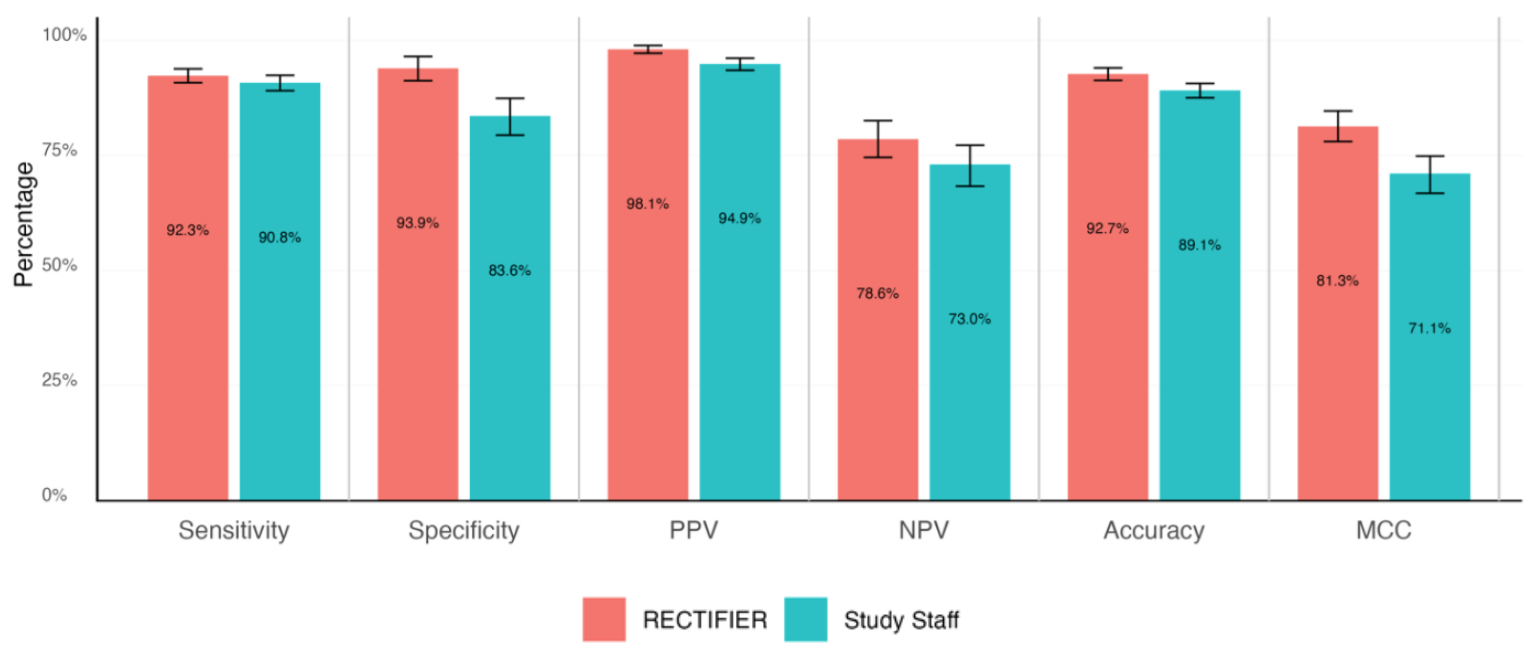
RECTIFIER performed as well, and in some cases better, than the study staff. Probably the most significant result of the study came from the cost comparison.
While no figures were given for study staff remuneration, it must have been significantly more than the cost of using GPT-4V which varied between $0.02 and $0.10 per patient. Using AI to evaluate a pool of 1,000 potential candidates would take a matter of minutes and cost about $100.
The researchers concluded that using an AI model like GPT-4V with RAG can maintain or improve accuracy in identifying clinical trial candidates, and do it more efficiently and much cheaper than using human staff.
They did note the need for caution in handing over medical care to automated systems, but it does seem that AI will do a better job than we can if properly directed.