AI revolves around data, but where does it come from? Are datasets legal and ethical? How do developers determine that for sure?
Training machine learning models such as large language models (LLM) requires large volumes of text data.
There are stacks of datasets available on platforms such as Kaggle, GitHub, and Hugging Face, but they exist in a legal and ethical grey area, primarily due to licensing and fair use issues.
The Data Provenance Initiative, a collaborative effort between AI researchers and legal professionals, reviewed thousands of datasets to shed light on their true origins.
It focused on over 1,800 data sets available on platforms, including Hugging Face, GitHub, and Papers With Code. The datasets are predominantly designed for fine-tuning open-source models such as Llama-2.
The study revealed that approximately 70% of these data sets either lacked clear licensing information or were tagged with overly permissive licenses.
With a glaring lack of clarity about copyright and commercial usage restrictions, AI developers risk accidentally breaking the law or violating copyright.
Shayne Longpre, a PhD candidate at MIT Media Lab who spearheaded the audit, emphasized that the issue is not the fault of hosting platforms but rather a systemic problem within the machine-learning community.
2023 has witnessed a deluge of lawsuits targeting major AI developers such as Meta, Anthropic, and OpenAI, who are under extreme pressure to adopt more transparent data collection practices. Regulations, such as the EU’s AI Act, are set to enforce precisely that.
The Data Provenance Initiative enables machine learning developers to explore the audited data sets here. The initiative also analyzes patterns within data sets, shedding light on their geographic and institutional origins.
Most datasets are constructed in the English-speaking Global North, highlighting sociocultural imbalances.
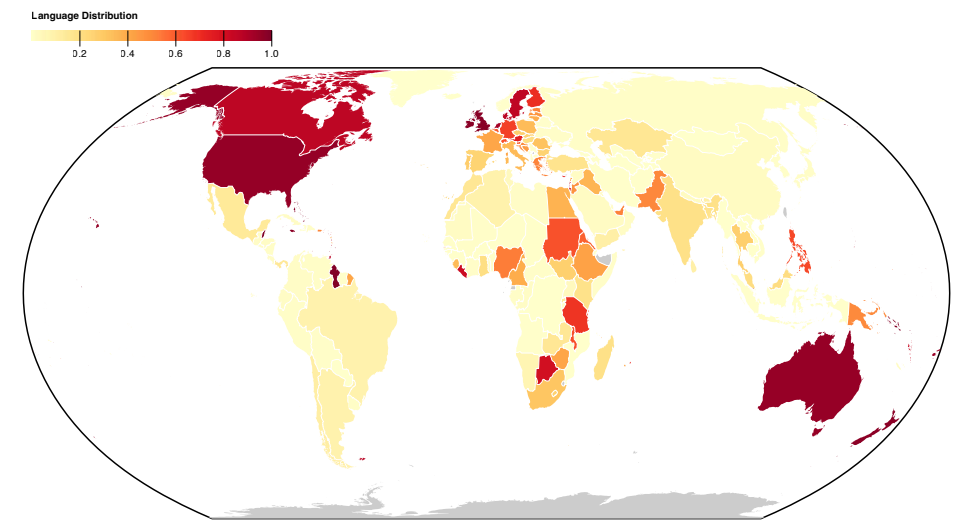
More about the study
This large-scale analysis of datasets uncovered systematic issues with how data is collected and distributed. The initiative also produced a paper to explain their findings, published here.
Here’s more information about the study’s methods and findings:
- Analyzing datasets for origin and labeling: This study systematically audited over 1800 fine-tuning datasets to scrutinize their data provenance, licensing, and documentation.
- Evidence of mislabeling: Findings highlighted the gap in data types available under different licenses and implications for legal interpretations of copyright and fair use. It unearthed a high rate of miscategorization of licenses, with over 72% of datasets not specifying a license and a 50% error rate in those that did.
- Unreliable data provenance: The research draws attention to the issue of unreliable data provenance, emphasizing the need for standards to trace data lineage, ensure proper attribution, and encourage responsible data use.
- Geographic distribution: The study highlights a serious lack of representation and attribution for datasets originating from the Global South. Most datasets revolve around the English language and are culturally bound to Europe, North America, and English-speaking Oceania.
This study highlights systemic and structural issues in how data is created, distributed, and used. Data is a critical resource for AI, and much like natural resources, it’s finite.
There is concern that AI technology will eventually outgrow current datasets and possibly even start consuming its own output, meaning AI models will learn from AI-generated text.
This could erode the quality of models, meaning high-quality, ethical, and legal data might become very valuable indeed.