AI is permeating the lives of millions worldwide, but despite widespread uptake, the technology is proving tricky to monetize amid escalating costs.
Generative AI tools like ChatGPT are costly to run, demanding high-end servers, expensive GPUs, and extensive ancillary hardware that guzzle vast power.
While largely unverified, Dylan Patel from SemiAnalysis told The Information that OpenAI allegedly forks out some $700,000 a day to run its models, posting losses of almost $500 million in 2022.
John Hennessy, chairman of Google’s parent company, Alphabet, said a single prompt on Bard costs up to 10 times more than a Google search, and analysts believe Google will incur billions in AI-related expenditure over the coming years.
2023 has acted as a testing ground for AI monetization. Tech giants like Microsoft, Google, and Adobe, among others, are attempting a wide variety of different approaches to create, promote, and price their AI offerings.
Most commercial models like ChatGPT, Bard, and Anthropic’s Claude 2 already restrict users to a certain number of prompts per hour or day, including paid versions like ChatGPT Plus. Adobe is setting monthly usage caps for its Firefly models.
AI’s immense costs are hitting enterprises, too. Adam Selipsky, the CEO of Amazon Web Services (AWS), commented on prohibitive costs for enterprise users wishing to build AI workloads, “A lot of the customers I’ve talked to are unhappy about the cost that they are seeing for running some of these models.”
Chris Young, Microsoft’s head of corporate strategy, noted that it’s still early days for enterprises looking to leverage current AI models, stating, “It will take time for companies and consumers to understand how they want to use AI and what they are willing to pay for it.”
He added, “We’re clearly at a place where now we’ve got to translate the excitement and the interest level into true adoption.”
AI models vs software
AI doesn’t offer the same economy as conventional software, as it requires specific computations for each task.
When users prompt a model like ChatGPT, it seeks patterns and sequences from its training data in the neural network architecture and calculates a specific answer. Each interaction draws energy, leading to ongoing costs.
Consequently, as adoption grows, so do expenses, posing a challenge to companies offering AI services at flat rates.
For example, Microsoft recently collaborated with OpenAI to introduce GitHub Copilot, aimed at programmers and developers.
Thus far, its high operational cost means it hasn’t been profitable. In the initial months of the year, Microsoft charged a subscription of $10 a month for this AI assistant and incurred an average loss of more than $20 a month for each user. Some users inflicted losses of up to $80 a month, as revealed by a source to the WSJ.
Microsoft’s Copilot suite for Microsoft 365 (confusingly named the same as the GitHub tool) plans to charge an extra $30 monthly. This tool offers capabilities like autonomously drafting emails, crafting PowerPoint presentations, and creating Excel spreadsheets.
Similarly, Google is also set to release an AI assistant feature for its workplace tools, imposing an additional $30 monthly charge on top of existing fees.
Both Microsoft and Google are banking on a flat monthly rate, hoping that the increased charges will adequately offset the average costs of powering these AI tools.
While $30/month might seem affordable in some more developed Western countries, these pricing models aren’t inclusive of much of the world population.
When ChatGPT Plus rolled out to the Indian market, for instance, many complained that it was far too expensive, costing a significant proportion of the average $330 monthly salary. Pricing billions of users out of AI may cost developers dearly when marketing tools to the public.
The cost is hardly immaterial to those living in more affluent countries, either, with surveys showing that subscriptions are among the first things to go when people seek to reduce their outgoings.
Why is AI so hard to monetize?
AI presents a void between developers’ seemingly infinite visions and the finite resources available to achieve them.
OpenAI CEO Sam Altman asserts that a futuristic AI-embedded world is simply ‘inevitable,’ where intelligent machines live beside us, doing our bidding, where people live for hundreds of years or immortally, and where AIs embedded in our brains help us perform complex tasks through us thinking alone.
He’s certainly not alone there, with Inflection CEO Mustafaya Suleman recently publishing his book “The Coming Wave,” where he compares AI to the Cambrian explosion 500 million years ago that led to the fastest burst in evolution ever witnessed on our planet.
Generative AI is set to explode, with revenue expected to exceed $1 trillion by 2032. From where it started to where it is now and where it will be is mind-blowing… every industry will be transformed for the better. pic.twitter.com/0licQGfphn
— Mustafa Suleyman (@mustafasuleyman) October 20, 2023
Ambitions fuel ideas, but money powers the tech industry. AI research and development is costly, so industry leaders, such as OpenAI, Google, and Facebook, invest heavily to stay ahead in the race.
AI companies attracted a whopping $94 billion investment in 2021, with multiple funding rounds reaching $500 million or more. The year 2023 upped the ante, with investments in startups Anthropic and Inflection passing the $1 billion mark.
AI specialists demand weighty salaries, often in the millions, due to fierce competition, and models require regular fine-tuning and stress-testing, adding to ongoing costs.
There are also ancillary costs relating to the data centers and maintenance, particularly cooling, as such hardware runs hot and is prone to breakdown if not kept at the optimum temperature.
In some circumstances, GPUs have short lifespans of under five years and frequently require specialist maintenance.
AI monetization strategies
In light of these challenges, how are tech companies approaching the challenge of AI monetization?
Enhancing productivity
Companies stand to benefit from AI by significantly boosting net societal productivity. By automating repetitive tasks, companies allow human professionals to focus on higher-level value-added functions.
This will help them tap into both private and public money. For example, DeepMind collaborates with the National Health Service (NHS) in the UK, and tech companies are working with governments on tackling climate change.
However, AI-driven productivity might impact tech companies’ other income streams. For example, Google’s ad revenues are declining as AI draws away traffic from its search engine. This year saw Google’s revenue from YouTube ads decline by 2.6%, and advertising network revenues decline by an unprecedented 8.3%.
Hardware sales
AI model training and hosting require specialized high-end hardware. GPUs are indispensable for running sophisticated AI algorithms, making them a lucrative component in the AI ecosystem.
Industry leader Nvidia is the big winner here, with its market cap hitting the $1 trillion mark, but other lower-key AI hardware manufacturers have benefitted, too.
Subscriptions
Offering AI enhancements as part of subscription packages is the go-to for generating revenue from public users and businesses who don’t want or need to go down the API route.
ChatGPT Plus is the most highly subscribed AI service globally, but information describing how much revenue it generates is lacking.
OpenAI launched its Enterprise variant this year to bolster subscription revenue and declared the company is on target for $1 billion in revenue over the next year.
APIs
Companies like OpenAI utilize a token-based billing approach for enterprise and business users for their APIs.
This system ensures that users are billed based on the actual computational load of their requests, ensuring fairness and transparency.
API pricing models cater to a broad spectrum of users, from those who make sporadic lightweight calls to heavy users with intensive tasks.
AI as an inherent feature
Some businesses are integrating AI capabilities into their products without immediately charging extra. This strategy aims to first boost a product’s inherent value.
Over time, once users have integrated the AI capabilities into their workflows and realized their value, they’re more likely to accept a subsequent price hike. However, AI has to deliver progressive advantages if costs steadily increase.
Open source AI training costs are getting cheaper
There is a parallel discussion here – why should anyone pay for AI when they never own it?
Some – like AI ‘godfather’ Yann LeCun – argue that AI should become part of our public infrastructure, emphasizing the need for developers to build open source models that are cheap and easy to access.
A NYT article on the debate around whether LLM base models should be closed or open.
Meta argues for openness, starting with the release of LLaMA (for non-commercial use), while OpenAI and Google want to keep things closed and proprietary.
They argue that openness can be…
— Yann LeCun (@ylecun) May 18, 2023
As AI solutions become integral to businesses, many don’t possess the budget to develop proprietary models from the ground up. Traditionally, they have turned to APIs from emerging AI startups or off-the-shelf systems.
However, as training costs diminish and the urge for data privacy grows, collaboration with vendors specializing in customizing both private and open-source models is increasingly favored.
Naveen Rao, CEO and co-founder of MosaicML, explained to The Register that open source models are tantalizing as they’re cheaper, more flexible, and enable businesses to keep workloads private.
MosaicML launched a series of open source large language models (LLMs) based on its MPT-7B architecture. Unlike many other LLMs, this model is commercially viable for the masses.
Rao conveyed the rationale behind this model, saying, “There’s definitely a lot of pull for this kind of thing, and we did it for several reasons.” He added, “One was we wanted to have a model out there that has permission for commercial use. We don’t want to stifle that kind of innovation.”
Addressing the affordability, Rao remarked, “If a customer came to us and said train this model, we can do it for $200,000 and we still make money on that.”
MosaicML also provides businesses with the tools to efficiently host their custom models on cloud platforms. “Their data is not shared with the startup, and they own the model’s weights and its IP,” confirmed Rao.
Rao also touched upon the limitations of commercial APIs, stating, “Commercial APIs are a great prototyping tool. I think with ChatGPT-type services people will use them for entertainment, and maybe some personal stuff, but not for companies. Data is a very important moat for companies.”
Rao further highlighted the costs of in-house architecture, stating, “GPUs actually fail quite often,” “If a node goes down, and there’s a manual intervention required, it took you five hours [to fix], you’ve just burned $10,000 with no work, right?”
He also addressed the looming issue of chip shortages in the industry, stating, “We’re going to live in this world of GPU shortages for at least two years, maybe five.”
If businesses can tap into open source AI to train their low-cost models with total data sovereignty and control, then that poses a challenge to public AI like ChatGPT.
AI’s looming bottlenecks?
In addition to the GPU shortages highlighted by Rao, another barrier to AI monetization is the industry’s rocketing energy requirements.
A recent study predicts that by 2027, the energy consumed by the AI industry might be equivalent to that of a small nation.
Microsoft’s rocketing data center water consumption affirms AI’s immense thirst for natural resources. Evidence indicates that recent spikes in water usage by tech giants like Microsoft and Google are attributed to intensive AI-related workloads.
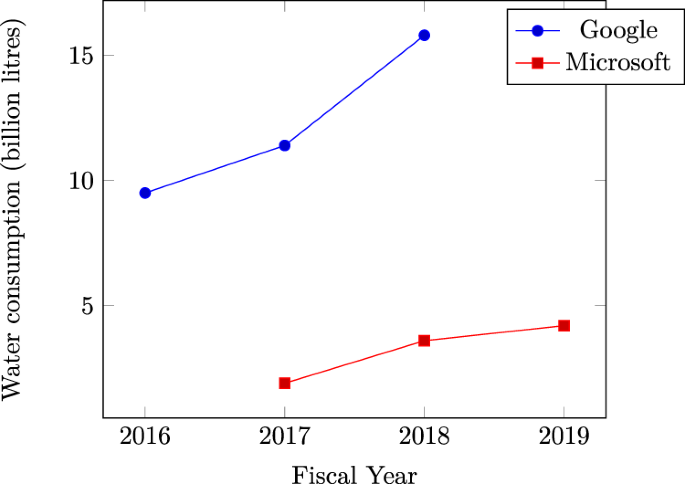
On a broader scale, the cumulative energy utilized by data centers already surpasses 1% of global electricity consumption, as per the International Energy Agency (IEA).
If energy demands continue to surge, then AI companies could be forced into turning their attention to developing more efficient approaches to model training, which might harm the technology’s near-term trajectory.
Competing narratives
The nascent AI industry remains young, and its future direction is exceptionally tough to predict.
Central figures in AI acknowledge that their preconceptions don’t always align with reality.
For instance, speaking on the Joe Rogan podcast, Sam Altman conceded that he was wrong about AI’s development course, describing the path to realizing highly intelligent forms of AI will be granular rather than explosive.
On the one hand, AI is proving challenging to monetize, casting doubts over whether the initial hype will subside, leading to a slower process of maturing the technology.
On the other hand, the industry may already have made enough progress to push AI towards a ‘singularity’ where it outpaces human cognition.
Over the next few years, if humanity scores progression in essential fields such as energy production and building low-power AI hardware and architecture – and progress has been made there this year – then the technology may obliterate near-term bottlenecks and continue its wicked pace of development.