From the foundations laid by Ada Lovelace and Charles Babbage to Alan Turing’s groundbreaking computing research, the world has been enthralled by the promise of AI – a dream to create machine-based entities possessing human-like cognitive abilities.
However, the trajectory of AI development later turned away from its biological roots in favor of brute-force computational power and algorithmic complexity.
With that, science fiction-infused dreams of lifelike robots have somewhat dwindled into a reality of more superficially mundane large language models (LLMs) like ChatGPT.
Of course, current AI models are still captivating, but they act as a tool rather than a being.
It’s still early days, but despite phenomenal advances, AI’s computational arms race has exposed gaps in our pursuit of genuinely intelligent machines.
No matter how powerful our algorithms become, they lack elegance, adaptability, and energy efficiency – the hallmarks of biological systems.
Researchers know this – and it frustrates them.
Professor Tony Prescott and Dr. Stuart Wilson from the University of Sheffield recently highlighted that most AI models, like ChatGPT, are ‘disembodied,’ meaning they lack a direct connection to the physical environment.
In contrast, the human brain has evolved within a physical system — our bodies — which enables us to directly sense and interact with the world.
Researchers are itching to free AIs from their monolithic architecture, which has led to a resurgence in bio-inspired AI, sometimes called neuromorphic AI, a sub-discipline that seeks to emulate the complex processes found in nature to create smarter, more efficient systems.
These efforts draw on various biological frameworks, from the structures that constitute our brains to swarm intelligence observed in ants or birds.
In the quest for autonomy and efficiency, bio-inspired AI forces us to examine long-standing computational problems, such as moving away from resource-heavy architectures built from thousands of power-hungry GPUs to lighter, more intricate analog systems.
Prescott, who co-authored a recent paper, “Understanding brain functional architecture through robotics,” emphasizes, “It is much more likely that AI systems will develop human-like cognition if they are built with architectures that learn and improve in similar ways as the human brain, using its connections to the real world.”
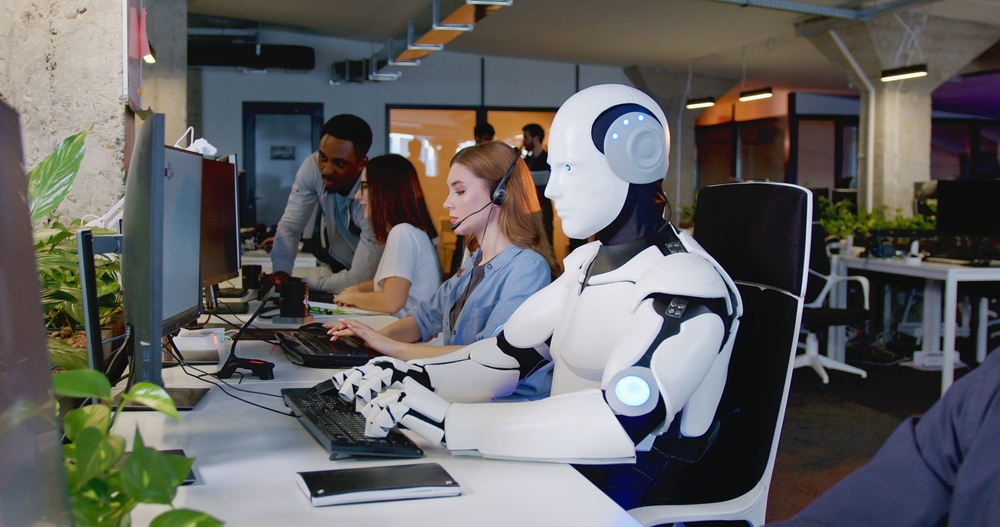
The human brain is a case in point – every thought and action your brain conjures requires only the power of a dim light bulb – about 20 watts.
And it goes further than that. Even when humans receive no external energy from food, they can survive for over a month. Extremophiles have found methods to thrive in some of the most inhospitable environments on the planet.
Compare that to the infrastructure required to power AI models like ChatGPT, which requires equivalent power to a small town and cannot self-replicate, heal, or adapt to its environment.
To give AI a fair hearing, you can argue that comparing AI to biologically intelligent systems is a flawed exercise.
After all, computers and brains simply excel at different tasks – it’s perhaps human nature to fuse them together in anthropomorphic visions of autonomous AIs that interact with the environment like the biological beings we’ve evolved beside.
However, AI researchers and neuroscientists alike are willing to fall into this intellectual deadlock, and many would describe the brain ‘as a computer’ that can be modeled and replicated artificially.
The EU’s Human Brain Project (HBP), a near-$1bn multinational experiment in Big Science, was a lesson in how the brain’s complexity evades artificial modeling.
The HBP set out to model the human brain in its entirety but only succeeded in modeling bits and pieces of its functionality.
Our brain – as a single entity – defeated the collective brains of thousands of researchers with vast funding and computing power at their fingertips – call it poetic justice.
As it happens, consciousness and the essence of thought formation is a similarly distant frontier to the far depths of space – we’re just not there yet.
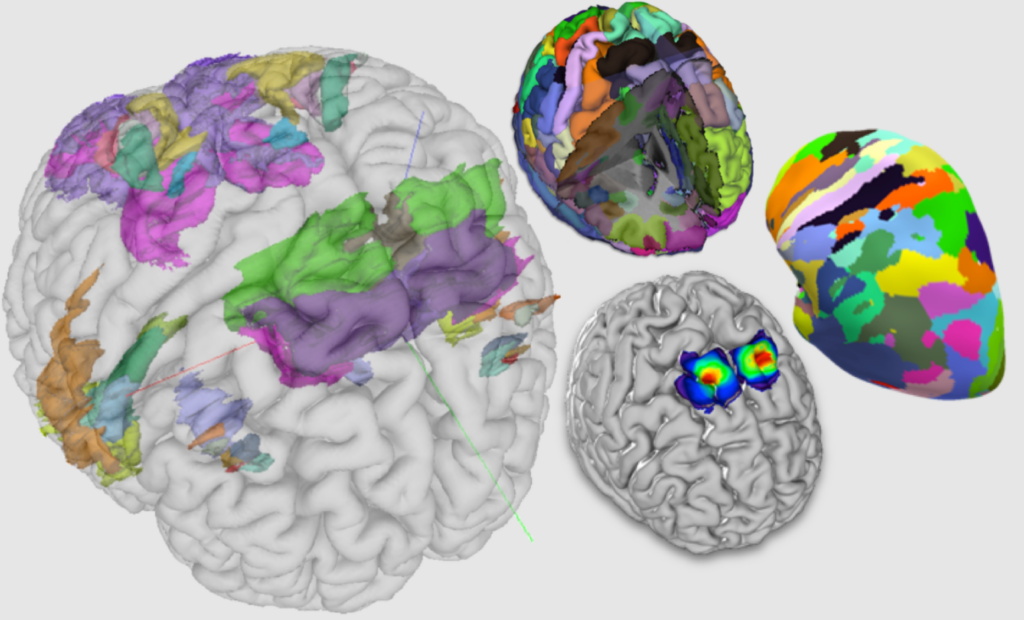
At the heart of this issue is the disconnect between biology and machines.
While neural networks and other forms of machine learning (ML) architecture are modeled via analogy to biological brains, the method of computation is fundamentally different.
Rodney Brooks, emeritus professor of robotics at MIT, reflected on this deadlock, stating, “There is a worry that his version of computation, based on functions of integers, is limited. Biological systems clearly differ. They must respond to varied stimuli over long periods of time; those responses in turn alter their environment and subsequent stimuli. The individual behaviors of social insects, for example, are affected by the structure of the home they build and the behavior of their siblings within it.”
Brooks sums up this paradox by asking, “Should those machines be modeled on the brain, given that our models of the brain are performed on such machines?”
The journey of bio-inspired AI
Nature has had millions of years of ‘R&D’ to perfect its incredibly resilient mechanisms.
The trend toward bio-inspired AI can be seen as a course correction, a humble acknowledgment that our quest for advanced AI might have led us down a path that, while still dazzling in its complexity, may be unsustainable in the long run.
Or at least, the current trajectory may not fulfill what humanity is ultimately seeking from AI. If we want to live in ‘the future’ where humans and robots walk side-by-side (though, of course, not everyone wants that), then we have to do better than amassing more GPUs and training larger models.
With that said, there is hope for the ardent futurists among us, as researchers have been mulching ideas of bio-inspired computing for decades, and some speculative ideas are beginning to find their feet.
In the late ’50s and early ’60s, Frank Rosenblatt’s work on the Perceptron offered the first simplified model of a biological neuron.
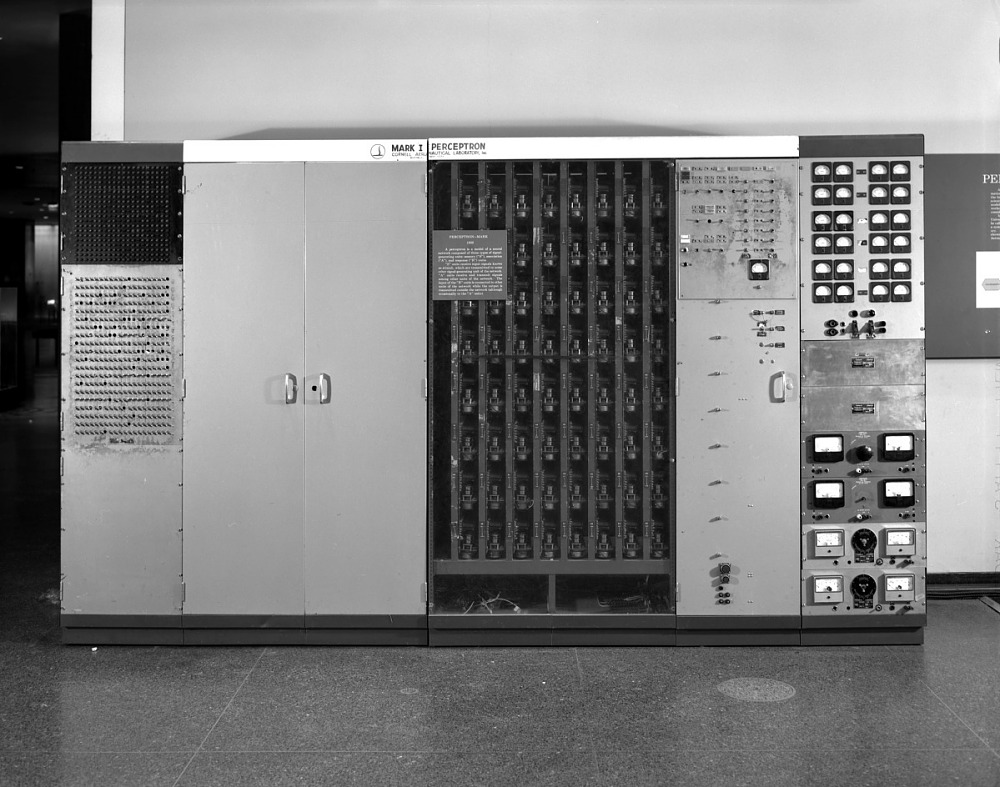
However, the 1986 paper “Learning representations by back-propagating errors” by David Rumelhart, Geoffrey Hinton, and Ronald Williams changed the game.
Now often referred to as the ‘godfather of neural networking (or AI in general),’ Hinton and his team introduced the backpropagation algorithm, providing a robust mechanism for training multi-layered neural networks, which propelled the field into applications ranging from natural language processing (NLP) to computer vision (CV) – two foundational branches of modern AI.
Soon after, bio-inspiration took a different route, borrowing from Darwinian principles. John Holland’s 1975 book “Adaptation in Natural and Artificial Systems” laid the groundwork for genetic algorithms.
By simulating mechanisms like mutation and natural selection, this approach unlocked a powerful tool for optimization problems, finding use in industries such as aerospace and finance.
Concepts such as ‘swarm intelligence,’ observed in swarms of insects and the synchronized movement of birds and fish, were first introduced into computing in the 80s and 90s and have seen notable advances in 2023.
In August 2023, ex-Google employees founded Sakana, a startup proposing to develop an ensemble of smaller AI models operating in concert.
Sakana’s approach is inspired by biological systems such as schools of fish or neural networks, where smaller units work together to achieve a more complex goal.
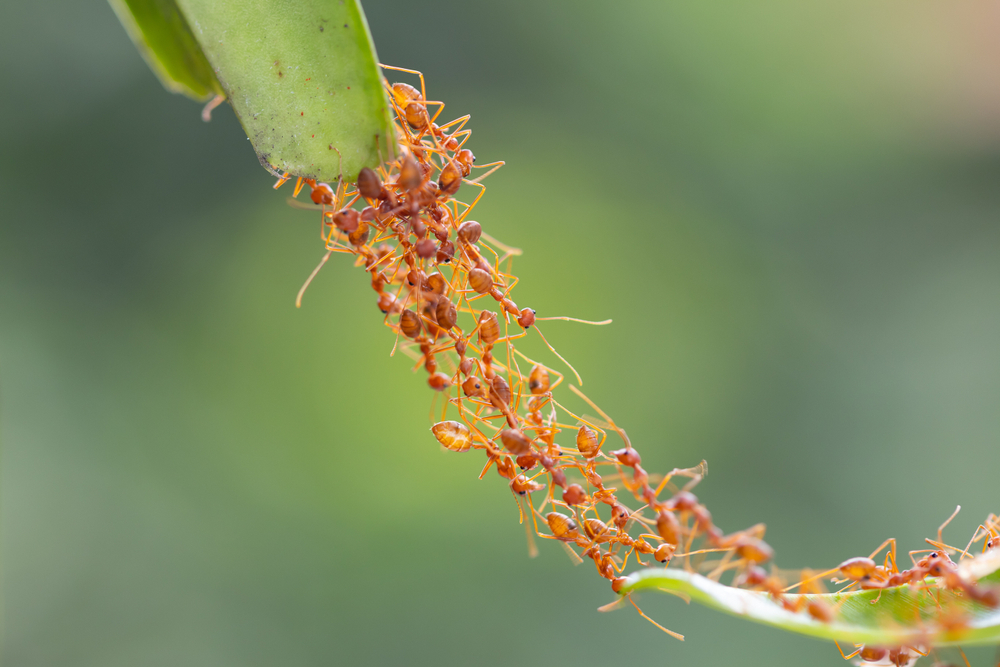
Acknowledging the monolithic architectures of modern AI models like ChatGPT, this ensembling approach promises to reduce power usage, and offers increased adaptability and resilience – qualities intrinsic to biological organisms.
Even reinforcement learning (RL), a branch of machine learning concerned with teaching algorithms to make decisions in pursuit of a reward, was largely bio-inspired.
Richard Sutton and Andrew Barto’s seminal book “Reinforcement Learning: An Introduction” draws numerous examples from how animals learn from their environment, inspiring algorithms that can adapt based on rewards and penalties.
The book makes hundreds of comparisons to animal behavior, citing, “Of all the forms of machine learning, reinforcement learning is the closest to the kind of learning that humans and other animals do.”
Towards bio-inspired AI
In complex biological beings like humans and other vertebrates, different components of the nervous system work in concert to manage a wide array of functions.
The central nervous system (CNS) serves as the control hub, processing information and orchestrating responses.
Meanwhile, the peripheral nervous system (PNS) acts as the communication network, transmitting signals between the CNS and other parts of the body.
Within the PNS is the specialized autonomic nervous system (ANS), which operates involuntarily to manage vital functions such as heart rate and digestion. Each system has its distinct roles, yet they are interconnected and collaborate seamlessly to help us navigate the environment.
Simpler organisms like insects have a slimmer, more economical nervous system, albeit still incredibly complex. A fruit fly has some 3,000 neurons and half a million synapses.
The components of the biological nervous system are anatomically distinct but work holistically, linked via neurons that send and receive sensory stimuli, ultimately forming a conceptual understanding – or consciousness in more complex beings.
To create autonomous robots with tightly coupled brains and sensory systems, researchers must move away from brute-force computing and create lightweight systems grounded in sensory reality.
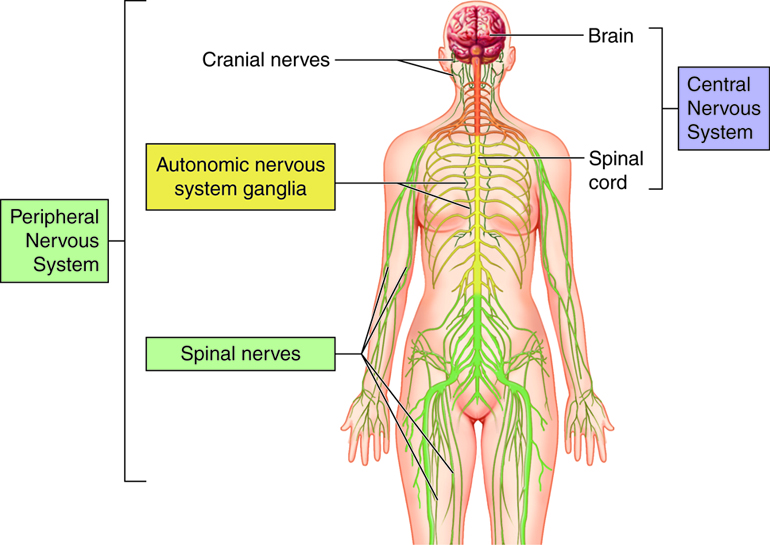
While AI models like ChatGPT have immense knowledge, they’re somewhat locked in time and locked out of sensory reality, with understandings primarily driven by their training data.
This does confer advantages, or rather, grants AI a distinguishable skillset to biological beings – which is perhaps why humanity is keen to develop AI to plug the inefficiencies of being a biological being.
As Amnon Shashua highlights, the “vastly different architecture of the computer favors strategies that make optimal use of its practically unlimited memory capacity and brute-force.”
However, if we’re ever to relinquish AI from the confines of data centers and web browsers, researchers must resolve these challenges and find ways of linking AI systems to a ‘body,’ or at least providing it with a robust sensory grounding.
This has immediate practical uses. Take the example of driverless cars – their sensory systems must function similarly to ours to work safely. Otherwise, they have no hope of ‘seeing’ a potential obstacle and reacting quickly to save disaster, which is proving a significant obstacle to their mass adoption.
In this vein, Dennis Bray, Department of Physiology, Development and Neuroscience, University of Cambridge, argued, “Machines can match us in many tasks, but they work differently from networks of nerve cells. If our aim is to build machines that are ever more intelligent and dexterous, then we should use circuits of copper and silicon. But if our aim is to reproduce the human brain, with its quirky brilliance, capacity for multitasking and sense of self, we have to look for other materials and different designs.”
These comments, though still relevant today, were published in a Nature discussion article published in 2012 for Turing’s centenary – and AI has evolved rapidly since then.
So where are we now?
Spiking neural networks (SNNs) and biological hardware
Researchers today are exploring the “other materials and different designs” Bray refers to, such as spiking neural networks (SNNs), a type of neural network intimately modeled on neuronic functionality.
SNNs offer a specialized alternative to the conventional neural networks we often encounter in machine learning.
Instead of relying on continuous activation functions to process input data, SNNs mimic the intricacies of biological neural networks by employing discrete spikes for inter-neuronal communication.
In these networks, each artificial neuron integrates incoming spikes from its connected neurons over time. When the accumulated signal, or membrane potential, surpasses a certain threshold, the neuron itself fires a spike.
This spiking mechanism allows the network to capture and process both spatial and temporal patterns, much like the neurons in biological brains.
So, what makes SNNs a focal point in bio-inspired AI?
Firstly, their ability to naturally process temporal data sequences sets them apart, eliminating the need for additional memory units like those seen in recurrent neural networks (RNNs)
Secondly, SNNs have been designed to be incredibly energy-efficient. Unlike traditional neural networks where each neuron is constantly active, the sparse and event-driven nature of SNNs allows for neurons to remain mostly inactive, firing spikes only when it’s essential. This significantly lowers their energy consumption.
Lastly, by mimicking biological systems more closely, SNNs have the potential for increased robustness and flexibility, especially in noisy or unpredictable settings.
Although the concept of SNNs has its roots in a theoretical understanding of biological neural systems, advances in hardware technology have made these networks more accessible for computational tasks.
Neuromorphic chips, specifically designed to efficiently simulate spiking dynamics, have played a significant role in making SNNs practically usable.
IBM’s bio-inspired analog chip and SNNs
The last two years have witnessed essential advancements in building ultra-light, energy-efficient AI solutions, also called neuromorphic chips.
Several other types of neuromorphic technologies are available now, too, such as neuromorphic cameras modeled on biological eyes.
Developed in 2023, IBM’s chip uses analog components like memristors to store varying numerical values. It also employs phase-change memory (PCM) to register a spectrum of values rather than 0s and 1s.
These attributes allow for reduced data transmission between memory and processor, providing an edge in energy efficiency. IBM’s design features “64 analog in-memory compute cores, each containing a 256-by-256 synaptic array.” It achieved an impressive 92.81% accuracy on a computer vision (CV) benchmark test while being over 15 times more efficient than several existing chips.
While IBM’s chip is not explicitly based on SNNs, the analog nature and the use of memristors make it highly compatible with the SNN model.
In essence, the SNNs could be implemented more naturally on this kind of architecture.
IIT Bombay’s SNN-based chip
In 2022, researchers from the Indian Institute of Technology, Bombay, designed a chip that works specifically with SNNs.
This chip uses band-to-band tunneling (BTBT) current for ultra-low-energy artificial neurons. According to Professor Udayan Ganguly, the chip achieves “5,000 times lower energy per spike at a similar area and 10 times lower standby power at a similar area and energy per spike.”
This type of chip has direct applications in compact devices like mobile phones, unmanned autonomous vehicles (UAVs), and IoT devices, fulfilling the need for lightweight and energy-efficient AI computing.
Both approaches aim to eventually enable what Ganguly describes as “an extremely low-power neurosynaptic core and developing a real-time on-chip learning mechanism, which are key for autonomous biologically inspired neural networks. This is the holy grail.”
These systems could combine the ‘system of thought’ with the ‘system of action and movement,’ similar to what we observe in biological organisms.
This would allow us to take a significant step toward creating artificial systems that are powerful, sustainable, and closely aligned with the biological systems that have inspired AI for almost a century.
At last, humanity could free AIs from monolithic architecture, unplug them from their power sources, and send them out into the world – and the universe – as autonomous beings.
Whether or not this is a good idea – well, that’s a discussion for another time.