As conversations swell around the risks of AI systems, we can’t overlook the strain technology places on the world’s already-taxed energy and water supplies.
Complex machine learning (ML) projects depend on a constellation of technologies, including training hardware (GPUs) and hardware for hosting and deploying AI models.
While efficient AI training techniques and architectures promise to reduce energy consumption, the AI boom has just started, and big tech is ramping up investment in resource-hungry data centers and cloud technology.
As the climate crisis deepens, striking a balance between technological advancement and energy efficiency is more critical than ever.
Energy challenges for AI
AI’s energy consumption has risen with the advent of complex, computationally expensive architectures such as neural networks.
For instance, GPT-4 is rumored to be based on 8 models with 220 billion parameters each, for a total of about 1.76 trillion parameters. Inflection is currently building a cluster of 22,000 high-end Nvidia chips, which could cost around $550,000,000 at a rough retail price of $25,000 per card. And that’s just for the chips only.
Each advanced AI model requires immense resources to train, but it’s been challenging to understand the true cost of AI development precisely until recently.
A 2019 study from the University of Massachusetts at Amherst investigated resource consumption associated with Deep Neural Networks (DNNs) approaches.
Typically, these DNNs require data scientists to manually design or use Neural Architecture Search (NAS) to find and train a specialized neural network from scratch for each unique case.
This approach is not only resource-intensive but also has a significant carbon footprint. The study discovered that training a single large Transformer-based neural network, built using NAS – a tool commonly employed in machine translation – generated around 626,000 pounds of carbon dioxide.
This is approximately equivalent to the lifetime gas emissions of 5 cars.
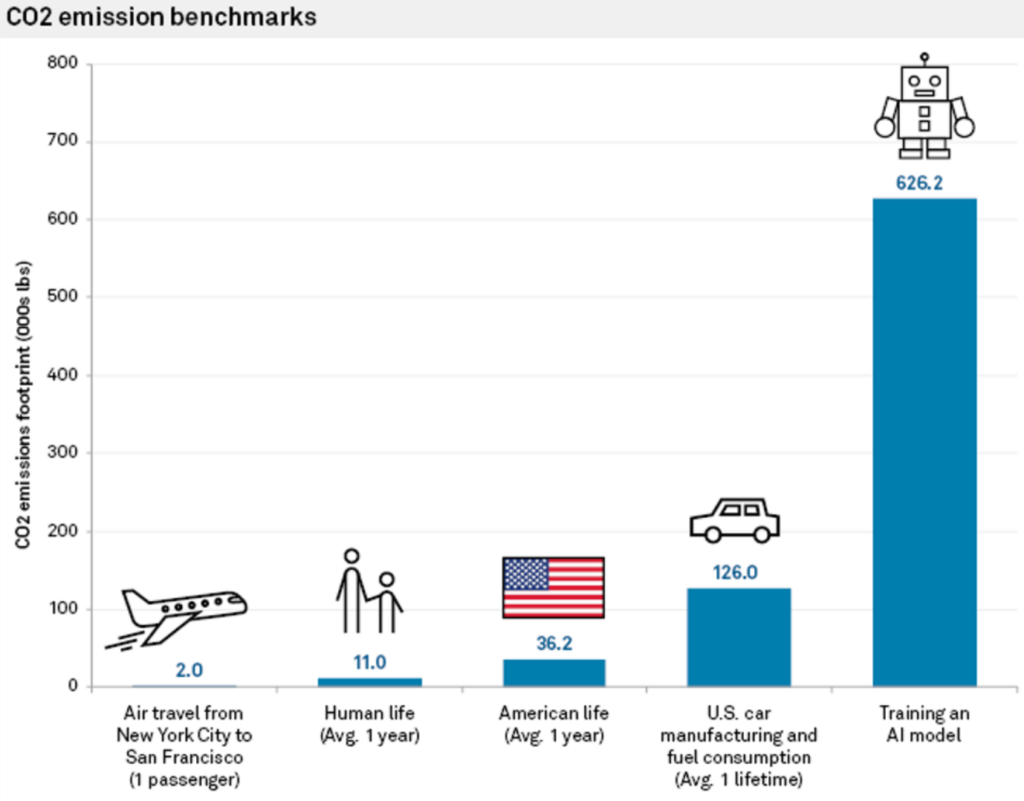
Carlos Gómez-Rodríguez, a computer scientist at the University of A Coruña in Spain, commented on the study, “While probably many of us have thought of this in an abstract, vague level, the figures really show the magnitude of the problem,” adding, “Neither I nor other researchers I’ve discussed them with thought the environmental impact was that substantial.”
The energy costs of training the model are only baselines – the minimum amount of required work to get a model operational.
As Emma Strubell, a Ph.D. candidate at the University of Massachusetts, says, “Training a single model is the minimum amount of work you can do.”
MIT’s ‘Once-For-All’ approach
Researchers at MIT later proposed a solution to this problem: the ‘Once-For-All’ (OFA) approach.
The researchers describe the issue with conventional neural network training: “Designing specialized DNNs for every scenario is engineer-expensive and computationally expensive, either with human-based methods or NAS. Since such methods need to repeat the network design process and retrain the designed network from scratch for each case, their total cost grows linearly as the number of deployment scenarios increases, which will result in excessive energy consumption and CO2 emissions.”
With MIT’s OFA paradigm, researchers train a single general-purpose neural network from which various specialized sub-networks can be created. The OFA process doesn’t require additional training for new subnetworks, reducing the energy-intensive GPU hours needed for model training and lowering CO2 emissions.
In addition to its environmental benefits, the OFA approach delivers substantial performance improvements. In internal tests, models created using the OFA approach performed up to 2.6 times faster on edge devices (compact IoT devices) than models created using NAS.
MIT’s OFA approach was recognized at the 4th Low Power Computer Vision Challenge in 2019 – an annual event hosted by the IEEE promoting research into enhancing the energy efficiency of computer vision (CV) systems.
The MIT team clinched top honors, with event organizers commending: “These teams’ solutions outperform the best solutions in literature.”
The 2023 Low Power Computer Vision Challenge is currently receiving submissions through to the 4th of August.
The role of cloud computing on AI’s environmental impact
In addition to training models, developers need immense cloud resources to host and deploy their models.
Big tech firms like Microsoft and Google are increasing investment in cloud resources over 2023 to handle the rising demands of AI-related products.
Cloud computing and its associated data centers have immense resource requirements. As of 2016, estimates suggested that data centers worldwide accounted for about 1% to 3% of global electricity consumption, equivalent to the energy usage of certain small nations.
The water footprint of data centers is also colossal. Large data centers can consume millions of gallons of water daily.
In 2020, it was reported that Google’s data centers in South Carolina were permitted to use 549 million gallons of water, nearly twice the amount used two years prior. A 15-megawatt data center can consume up to 360,000 gallons of water daily.
In 2022, Google disclosed that its global data center fleet consumed around 4.3 billion gallons of water. However, they highlight that water cooling is substantially more efficient than other techniques.
Big tech companies all have similar plans for reducing their resource use, such as Google, which achieved their goal of matching 100% of their energy use with renewable energy purchases in 2017.
Next-gen AI hardware modeled on the human brain
AI is immensely resource-intensive, but our brains run off a mere 12 watts of power – can such power efficiency be replicated in AI technology?
Even a desktop computer draws over 10x more power than the human brain, and powerful AI models require millions of times more power. Building AI tech that can replicate the efficiency of biological systems would completely transform the industry.
To be fair to AI, this comparison doesn’t account for the fact that the human brain was ‘trained’ over millions of years of evolution. Plus, AI systems and biological brains excel at different tasks.
Even so, building AI hardware that can process information with similar energy consumption to biological brains would enable autonomous biologically-inspired AIs that aren’t coupled to bulky power sources.
In 2022, a team of researchers from the Indian Institute of Technology, Bombay, announced the development of a new AI chip modeled on the human brain. The chip works with spiking neural networks (SNNs), which mimic the neural signal processing of biological brains.
The brain comprises 100 billion tiny neurons connected to thousands of other neurons via synapses, transmitting information through coordinated patterns of electrical spiking. The researchers built ultra-low-energy artificial neurons, equipping SNNs with band-to-band tunneling (BTBT) current.
“With BTBT, quantum tunneling current charges up the capacitor with ultralow current, meaning less energy is required,” explained Udayan Ganguly from the research team.
According to Professor Ganguly, compared to existing state-of-the-art neurons implemented in hardware SNNs, their approach achieves “5,000 times lower energy per spike at a similar area and 10 times lower standby power at a similar area and energy per spike.”
The researchers successfully demonstrated their approach in a speech recognition model inspired by the brain’s auditory cortex. SNNs could enhance applications on compact devices like mobile phones and IoT sensors.
The team aims to develop an “extremely low-power neurosynaptic core and a real-time on-chip learning mechanism, which are key for autonomous biologically inspired neural networks.”
AI’s environmental impacts are often overlooked, but solving problems such as power consumption for AI chips will also unlock new avenues of innovation.
If researchers can model AI technology on biological systems, which are exceptionally energy-efficient, this would enable the development of autonomous AI systems that don’t depend on ample power supply and data center connectivity.