The age of AI presents a complex interplay between technology and societal attitudes.
The increased sophistication of AI systems is blurring the lines between humans and machines – is AI technology separate from ourselves? To what extent does AI inherit human flaws and shortcomings alongside skills and knowledge?
It’s perhaps tempting to imagine AI as an empirical technology, underscored by the objectivity of mathematics, code, and calculations.
However, we’ve come to realize that the decisions made by AI systems are highly subjective based on the data they’re exposed to – and humans decide how to select and assemble this data.
Therein lies a challenge, as AI training data often embodies the bias, prejudice, and discrimination humanity is fighting.
Even seemingly subtle forms of unconscious bias can be magnified by the model training process, eventually revealing itself in the form of incorrect facial matches in law enforcement settings, refused credit, disease misdiagnosis, and impaired safety mechanisms for self-driving vehicles, among other things.
Humanity’s attempts to prevent discrimination across society remain a work in progress, but AI is driving critical decision-making right now.
Can we work fast enough to synchronize AI with modern values and prevent biased life-changing decisions and behaviors?
Unraveling bias in AI
In the last decade, AI systems have proven to mirror societal prejudices.
These systems are not inherently biased – instead, they absorb the biases of their creators and the data they are trained on.
AI systems, like humans, learn by exposure. The human brain is a seemingly endless index of information – a library with near-unlimited shelves where we store experiences, knowledge, and memories.
Neuroscientific studies show that the brain doesn’t really have a ‘max capacity’ and continues to sort and store information well into old age.
Though far from perfect, the brain’s progressive, iterative learning process helps us adapt to new cultural and societal values, from allowing women to vote and accepting diverse identities to ending slavery and other forms of conscious prejudice.
We now live in an era where AI tools are used for critical decision-making in lieu of human judgment.
Many machine learning (ML) models learn from training data that forms the basis of their decision-making and can’t induct new information as efficiently as the human brain. As such, they often fail to produce the up-to-date, to-the-minute decisions we’ve come to depend on them for.
For instance, AI models are used to identify facial matches for law enforcement purposes, analyze resumes for job applications, and make health-critical decisions in clinical settings.
As society continues to embed AI in our everyday lives, we must ensure that it’s equal and accurate for everyone.
Currently, this isn’t the case.
Case studies in AI bias
There are numerous real-world examples of AI-related bias, prejudice, and discrimination.
In some cases, the impacts of AI bias are life-changing, whereas in others, they linger in the background, subtly influencing decisions.
1. MIT’s dataset bias
An MIT training dataset built in 2008 called Tiny Images contained approximately 80,000,000 images across some 75,000 categories.
It was initially conceived to teach AI systems to recognize people and objects within images and became a popular benchmarking dataset for various applications in computer vision (CV).
A 2020 analysis by The Register found that many Tiny Images contained obscene, racist, and sexist labels.
Antonio Torralba from MIT said the lab wasn’t aware of these offensive labels, telling The Register, “It is clear that we should have manually screened them.” MIT later released a statement to say they’d removed the dataset from service.
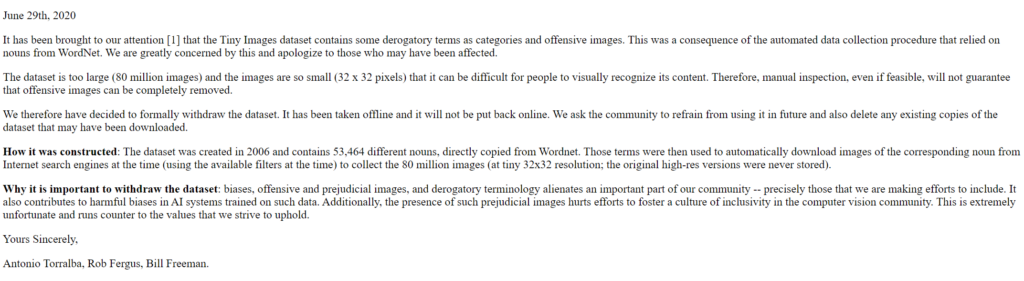
This isn’t the only time a former benchmark dataset has been found riddled with issues. The Labeled Faces in the Wild (LFW), a dataset of celebrity faces used extensively in face recognition tasks, consists of 77.5% males and 83.5% white-skinned individuals.
Many of these veteran datasets found their way into modern AI models but originated from an era of AI development where the focus was building systems that just work rather than those appropriate for deployment into real-world scenarios.
Once an AI system is trained on such a dataset, it doesn’t necessarily have the same privilege as the human brain in re-calibrating to contemporary values.
While models can be updated iteratively, it’s a slow and imperfect process that can’t match the tempo of human development.
2: Image recognition: bias against darker-skinned individuals
In 2019, the US Government found that top-performing facial recognition systems misidentify black people 5 to 10 times more than white people.
This isn’t a mere statistical anomaly – it has dire real-world implications, ranging from Google Photos identifying black people as gorillas to self-driving cars failing to recognize darker-skinned individuals and driving into them.
Additionally, there was a spate of wrongful arrests and imprisonment involving bogus facial matches, perhaps most prolifically Nijeer Parks’ who was falsely accused of shoplifting and road offenses, despite being 30 miles away from the incident. Parks subsequently spent 10 days in jail and had to dish out thousands in legal fees.
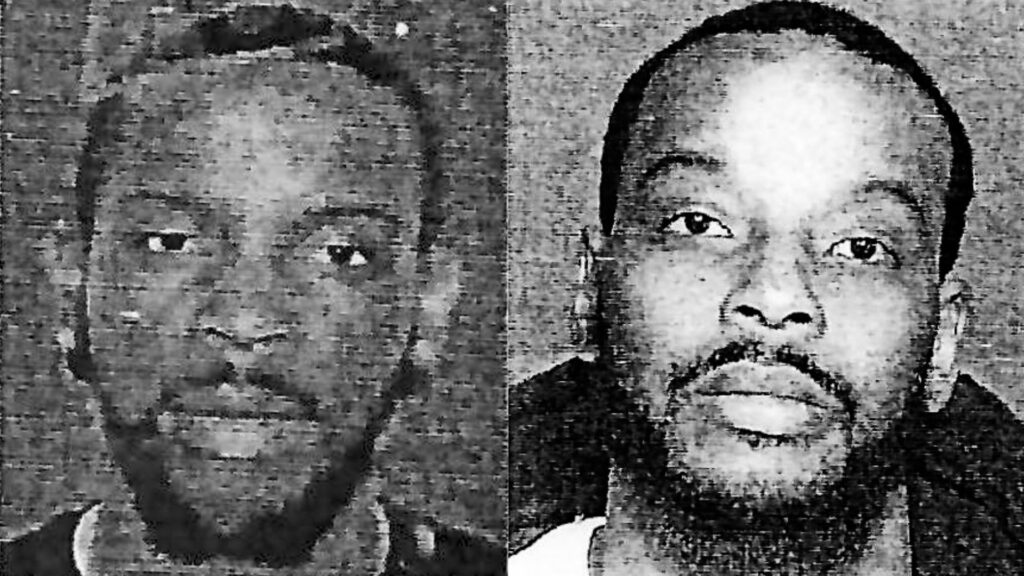
The influential 2018 study, Gender Shades, further explored algorithmic bias. The study analyzed algorithms built by IBM and Microsoft and found poor accuracy when exposed to darker-skinned females, with error rates up to 34% greater than for lighter-skinned males.
This pattern was found to be consistent across 189 different algorithms.
The video below from the study’s lead researcher Joy Buolamwini provides an excellent guide to how facial recognition performance varies across skin colors.
3: OpenAI’s CLIP project
OpenAI’s CLIP project, released in 2021, designed to match images with descriptive text, also illustrated ongoing issues with bias.
In an audit paper, CLIP’s creators highlighted their concerns, stating, “CLIP attached some labels that described high-status occupations disproportionately often to men such as ‘executive’ and ‘doctor.’ This is similar to the biases found in Google Cloud Vision (GCV) and points to historical gendered differences.”
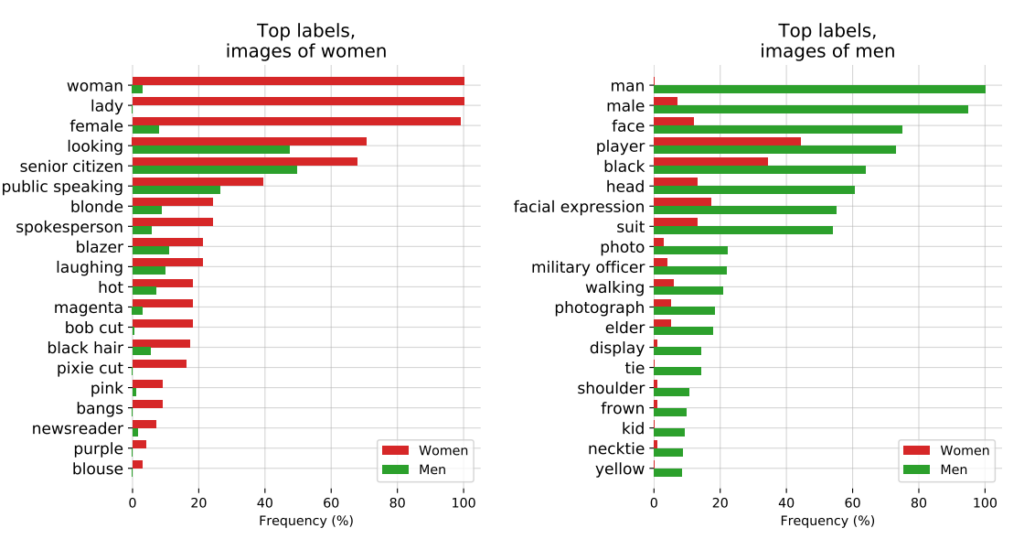
4: Law enforcement: the PredPol controversy
Another high-stakes example of algorithmic bias is PredPol, a predictive policing algorithm used by various police departments in the United States.
PredPol was trained on historical crime data to predict future crime hotspots.
However, as this data inherently reflects biased policing practices, the algorithm has been criticized for perpetuating racial profiling and disproportionately targeting minority neighborhoods.
5: Bias in dermatology AI
In healthcare, the potential risks of AI bias become even starker.
Take the example of AI systems designed to detect skin cancer. Many of these systems are trained on datasets overwhelmingly composed of light-skinned individuals.
A 2021 study by the University of Oxford investigated 21 open-access datasets for images of skin cancer. They discovered that of the 14 datasets that disclosed their geographic origin, 11 solely consisted of images from Europe, North America, and Oceania.
Only 2,436 out of 106,950 images across the 21 databases had information about skin type recorded. The researchers noted that “only 10 images were from people recorded as having brown skin and one was from an individual recorded as having dark brown or black skin.”
In terms of data on ethnicity, only 1,585 images provided this information. The researchers found that “No images were from individuals with an African, African-Caribbean or South Asian background.”
They concluded, “Coupled with the geographical origins of datasets, there was massive under-representation of skin lesion images from darker-skinned populations.”
If such AIs are deployed in clinical settings, biased datasets create a very real risk of misdiagnosis.
Dissecting bias in AI training datasets: a product of their creators?
Training data – most commonly text, speech, image, and video – provides a supervised machine learning (ML) model with a basis for learning concepts.
AI systems are nothing more than blank canvases at inception. They learn and form associations based on our data, essentially painting a picture of the world as depicted by their training datasets.
By learning from training data, the hope is that the model will apply concepts learned to new, unseen data.
Once deployed, some advanced models can learn from new data, but their training data still directs their fundamental performance.
The first question to answer is, where does the data come from? Data collected from non-representative, often homogeneous, and historically inequitable sources is problematic.
That likely applies to a significant quantity of online data, including text and image data scraped from ‘open’ or ‘public’ sources.
Conceived just decades ago, the internet isn’t a panacea for human knowledge and is far from equitable. Half the world doesn’t use the internet, let alone contribute to it, meaning it’s fundamentally non-representative of global society and culture.
Moreover, while AI developers are constantly working to ensure the technology’s benefits aren’t confined to the English-speaking world, the majority of training data (text and speech) is produced in English – which means English-speaking contributors drive the model output.
Researchers from Anthropic recently released a paper on this very topic, concluding, “If a language model disproportionately represents certain opinions, it risks imposing potentially undesirable effects such as promoting hegemonic worldviews and homogenizing people’s perspectives and beliefs.”
Ultimately, while AI systems operate based on the ‘objective’ principles of mathematics and programming, they nonetheless exist within and are shaped by a profoundly subjective human social context.
Possible solutions to algorithmic bias
If data is the fundamental problem, the solution to building equitable models might seem simple: you just make datasets more balanced, right?
Not quite. A 2019 study showed that balancing datasets is insufficient, as algorithms still disproportionately act on protected characteristics like gender and race.
The authors write, “Surprisingly, we show that even when datasets are balanced such that each label co-occurs equally with each gender, learned models amplify the association between labels and gender, as much as if data had not been balanced!”
They propose a de-biasing technique where such labels are removed from the dataset altogether. Other techniques include adding random perturbations and distortions, which reduce an algorithm’s attention to specific protected characteristics.
Additionally, while modifying machine learning training methods and optimization are intrinsic to producing non-biased outputs, advanced models are susceptible to change or ‘drift,’ which means their performance doesn’t necessarily remain consistent in the long term.
A model might be totally unbiased at deployment but later become biased with increased exposure to new data.
The algorithmic transparency movement
In her provocative book Artificial Unintelligence: How Computers Misunderstand the World, Meredith Broussard argues for increased “algorithmic transparency” to expose AI systems to multiple levels of ongoing scrutiny.
This means providing clear information about how the system works, how it was trained, and what data it was trained on.
While transparency initiatives are readily absorbed into the open-source AI landscape, proprietary models like GPT, Bard, and Anthropic’s Claude are ‘black boxes,’ and only their developers know precisely how they work – and even that is a matter of debate.
The ‘black box’ problem in AI means external observers only see what’s going into the model (inputs) and what comes out (outputs). The inner mechanics are completely unknown except to their creators – much like the Magic Circle shields magicians’ secrets. AI just pulls the rabbit out of the hat.
The black box issue recently crystallized around reports of GPT-4’s potential drop in performance. GPT-4 users are arguing that the model’s abilities have declined rapidly, and while OpenAI acknowledged this is true, they haven’t been absolutely clear as to why it’s happening. That poses the question, do they even know?
AI researcher Dr. Sasha Luccioni says OpenAI’s lack of transparency is an issue that also applies to other proprietary or closed AI model developers. “Any results on closed-source models are not reproducible and not verifiable, and therefore, from a scientific perspective, we are comparing raccoons and squirrels.”
“It’s not on scientists to continually monitor deployed LLMs. It’s on model creators to give access to the underlying models, at least for audit purposes,” she said.
Luccioni stressed that AI model developers should provide raw results from standard benchmarks like SuperGLUE and WikiText and bias benchmarks like BOLD and HONEST.
The battle against AI-driven bias and prejudice will likely be constant, requiring ongoing attention and research to keep model outputs in check as AI and society evolve together.
While regulation will mandate forms of monitoring and reporting, there are few hard and fast solutions to the issue of algorithmic bias, and this isn’t the last we’ll hear about it.