A new study shows that training AI image generators with AI-generated images eventually leads to a significant reduction in output quality.
Baraniuk and his team demonstrated how this problematic AI training loop affects generative AIs, including StyleGAN and diffusion models. These are among the models used for AI image generators like Stable Diffusion, DALL-E, and MidJourney.
In their experiment, the team trained the AIs on either AI-generated or real images. 70,000 real human faces sourced from Flickr.
When each AI was trained on its own AI-generated images, the StyleGAN image generator’s outputs began to display distorted and wavy visual patterns, while the diffusion image generator’s outputs became blurrier.
In both cases, training AIs on AI-generated images resulted in a loss of quality.
One of the study authors, Richard Baraniuk from Rice University in Texas, warns, “There’s going to be a slippery slope to using synthetic data, either wittingly or unwittingly.”
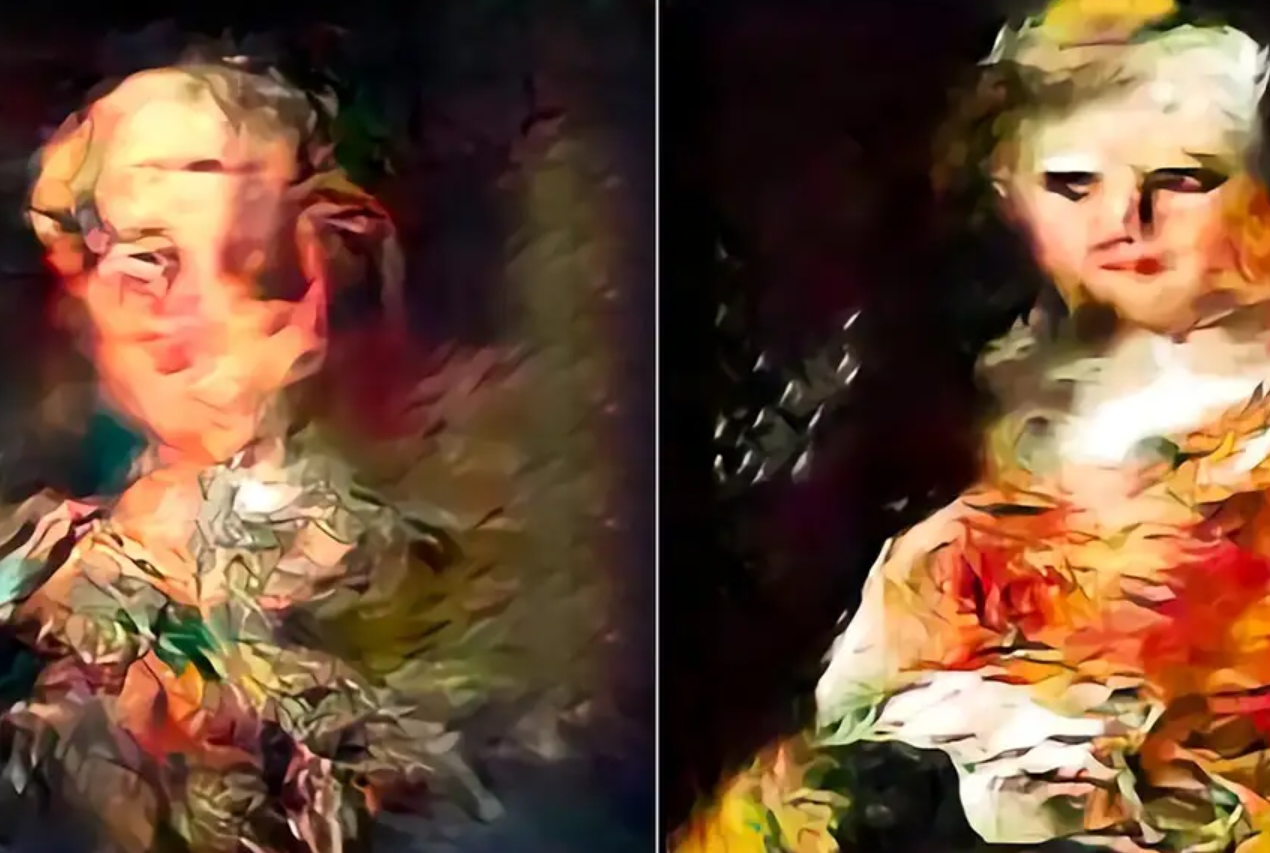
Although the decline in image quality was reduced by selecting higher-quality AI-generated images for training, this led to a loss of image diversity.
The researchers also tried incorporating a fixed set of real images into training sets that primarily included AI-generated images, a method sometimes used to supplement small training sets.
However, this only delayed the decline in image quality – it seems inevitable that the more AI-generated data enters training datasets, the worse the output becomes. It’s just a matter of when.
Reasonable results were achieved when each AI was trained on a mix of AI-generated images and a constantly changing set of authentic images. This helped maintain the quality and diversity of the images.
It’s challenging to balance quantity with quality – synthetic images are potentially unlimited compared to real images, but using them comes at a cost.
AIs are running out of data
AIs are data-hungry but authentic, high-quality data is a finite resource.
The findings in this research echo similar studies for text generation, where AI outputs tend to suffer when models are trained on AI-generated text.
The researchers highlight that smaller organizations with limited ability to collect authentic data face the greatest challenges in filtering AI-generated images from their datasets.
Additionally, the issue is compounded by the internet becoming inundated with AI-generated content, making it stunningly tricky to determine the type of data models are trained on.
Sina Alemohammad, from Rice University, suggests that developing watermarks to identify AI-generated images could help but warns that overlooked hidden watermarks can degrade the quality of AI-generated images.
Alemohammad concludes, “You are damned if you do and damned if you don’t. But it’s definitely better to watermark the image than not.”
The long-term consequences of AI consuming its output are hotly debated, but right now, AI developers need to find solutions to ensure the quality of their models.