Medical imaging is a complex field where interpreting results can be challenging.
AI models can assist doctors by analyzing images that might indicate disease-indicating anomalies.
However, there’s a catch: these AI models usually come up with a single solution when, in reality, medical images often have multiple interpretations.
If you ask five experts to outline an area of interest, like a small lump in a lung scan, you might end up with five different drawings, as they could all have their own opinions on where the lump starts and ends, for example.
To tackle this problem, researchers from MIT, the Broad Institute of MIT Harvard, and Massachusetts General Hospital have created Tyche, an AI system that embraces the ambiguity in medical image segmentation.
Segmentation involves labeling specific pixels in a medical image that represent important structures, like organs or cells.
Marianne Rakic, an MIT computer science PhD candidate and lead author of the study, explains, “Having options can help in decision-making. Even just seeing that there is uncertainty in a medical image can influence someone’s decisions, so it is important to take this uncertainty into account.”
Named after the Greek goddess of chance, Tyche generates multiple possible segmentations for a single medical image to capture ambiguity.
Each segmentation highlights slightly different regions, allowing users to choose the most suitable one for their needs.
Rakic tells MIT News, “Outputting multiple candidates and ensuring they are different from one another really gives you an edge.”
So, how does Tyche work? Let’s break it down into four simple steps:
- Learning by example: Users give Tyche a small set of example images, called a “context set,” that show the segmentation task they want to perform. These examples can include images segmented by different human experts, helping the model understand the task and the potential for ambiguity.
- Neural network tweaks: The researchers modified a standard neural network architecture to allow Tyche to handle uncertainty. They adjusted the network’s layers so that the potential segmentations generated at each step could “communicate” with each other and the context set examples.
- Multiple possibilities: Tyche is designed to output multiple predictions based on a single medical image input and the context set.
- Rewarding quality: The training process was tweaked to reward Tyche for producing the best possible prediction. If the user asks for five predictions, they can see all five medical image segmentations produced by Tyche, even if one might be better.
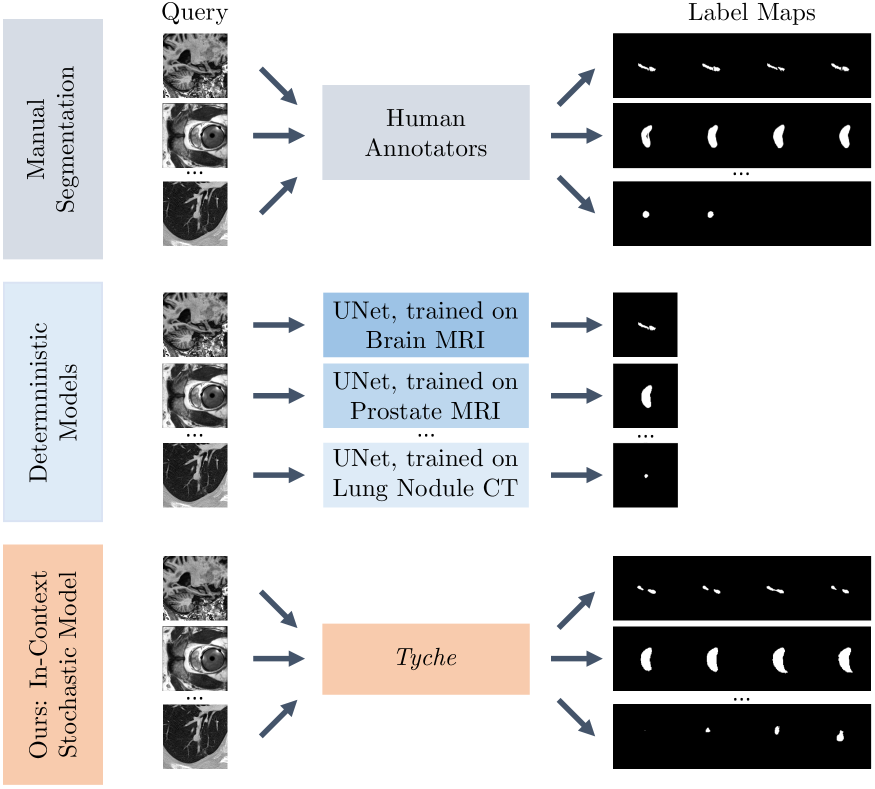
One of Tyche’s biggest strengths is its adaptability. It can take on new segmentation tasks without needing to be retrained from scratch.
Normally, AI models for medical image segmentation use neural networks that require extensive training on large datasets and machine learning expertise.
In contrast, Tyche can be used “out of the box” for various tasks, from spotting lung lesions in X-rays to identifying brain abnormalities in MRIs.
Numerous studies have been conducted in AI medical imaging, including major breakthroughs in breast cancer screening and AI diagnostics that match or even beat doctors in interpreting images.
Looking to the future, the research team plans to explore using more flexible context sets, possibly including text or multiple types of images.
They also want to develop ways to improve Tyche’s worst predictions and enable the system to recommend the best segmentation candidates.