A team of researchers from New York University has made progress in neural speech decoding, bringing us closer to a future in which individuals who have lost the ability to speak can regain their voice.
The study, published in Nature Machine Intelligence, presents a novel deep learning framework that accurately translates brain signals into intelligible speech.
People with brain injuries from strokes, degenerative conditions, or physical trauma can use these systems to communicate by decoding their thoughts or intended speech from neural signals.
The NYU team’s system involves a deep learning model that maps the electrocorticography (ECoG) signals from the brain to speech features, such as pitch, loudness, and other spectral content.
The second stage involves a neural speech synthesizer that converts the extracted speech features into an audible spectrogram, which can then be transformed into a speech waveform.
That waveform can finally be converted into natural-sounding synthesized speech.
New paper out today in @NatMachIntell, where we show robust neural to speech decoding across 48 patients. https://t.co/rNPAMr4l68 pic.twitter.com/FG7QKCBVzp
— Adeen Flinker 🇮🇱🇺🇦🎗️ (@adeenflinker) April 9, 2024
How the study works
This study involves training an AI model that can power a speech synthesis device, enabling those with speech loss to talk using electrical impulses from their brain.
Here’s how it works in more detail:
1. Gathering brain data
The first step involves collecting the raw data needed to train the speech-decoding model. The researchers worked with 48 participants who were undergoing neurosurgery for epilepsy.
During the study, these participants were asked to read hundreds of sentences aloud while their brain activity was recorded using ECoG grids.
These grids are placed directly on the brain’s surface and capture electrical signals from the brain regions involved in speech production.
2. Mapping brain signals to speech
Using speech data, the researchers developed a sophisticated AI model that maps the recorded brain signals to specific speech features, such as pitch, loudness, and the unique frequencies that make up different speech sounds.
3. Synthesizing speech from features
The third step focuses on converting the speech features extracted from brain signals back into audible speech.
The researchers used a special speech synthesizer that takes the extracted features and generates a spectrogram—a visual representation of the speech sounds.
4. Evaluating the results
The researchers compared the speech generated by their model to the original speech spoken by the participants.
They used objective metrics to measure the similarity between the two and found that the generated speech closely matched the original’s content and rhythm.
5. Testing on new words
To ensure that the model can handle new words it hasn’t seen before, certain words were intentionally left out during the model’s training phase, and then the model’s performance on these unseen words was tested.
The model’s ability to accurately decode even new words demonstrates its potential to generalize and handle diverse speech patterns.
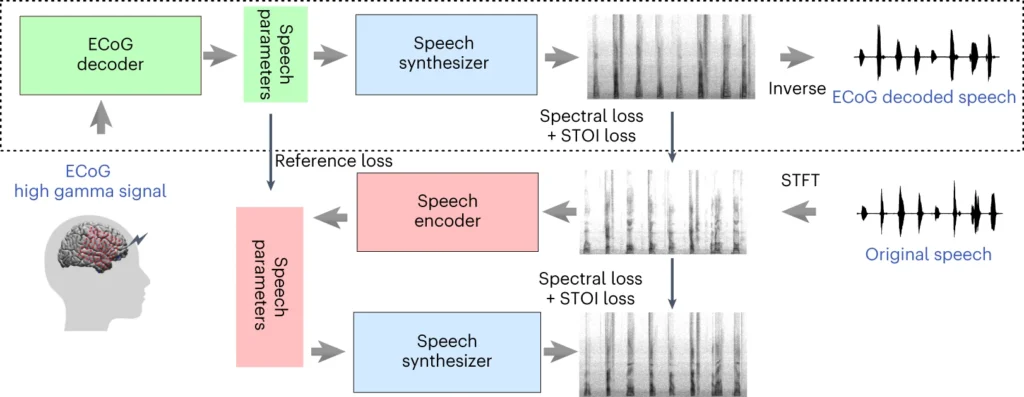
The top section of the above diagram describes a process for converting brain signals to speech. First, a decoder turns these signals into speech parameters over time. Then, a synthesizer creates sound pictures (spectrograms) from these parameters. Another tool changes these pictures back into sound waves.
The bottom section discusses a system that helps train the brain signal decoder by mimicking speech. It takes a sound picture, turns it into speech parameters, and then uses those to make a new sound picture. This part of the system learns from actual speech sounds to improve.
After training, only the top process is needed to turn brain signals into speech.
One key advantage of NYU’s system is its ability to achieve high-quality speech decoding without the need for ultra-high-density electrode arrays, which are impractical for long-term use.
In essence, it offers a more lightweight, portable solution.
Another achievement is the successful decoding of speech from both the left and right hemispheres of the brain, which is important for patients with brain damage on one side of the brain.
Converting thoughts to speech using AI
The NYU study builds upon previous research in neural speech decoding and brain-computer interfaces (BCIs).
In 2023, a team at the University of California, San Francisco, enabled a paralyzed stroke survivor to generate sentences at a speed of 78 words per minute using a BCI that synthesized both vocalizations and facial expressions from brain signals.
Other recent studies have explored the use of AI to interpret various aspects of human thought from brain activity. Researchers have demonstrated the ability to generate images, text, and even music from MRI and electroencephalogram (EEG) data taken from the brain.
For example, a study from the University of Helsinki used EEG signals to guide a generative adversarial network (GAN) in producing facial images that matched participants’ thoughts.
Meta AI also developed a technique for partially decoding what someone was listening to using brainwaves collected non-invasively.
Opportunities and challenges
NYU’s method uses more widely available and clinically viable electrodes than past methods, making it more accessible.
While this is exciting, there are major obstacles to overcome if we’re to witness widespread use.
For one, collecting high-quality brain data is a complex and time-consuming endeavour. Individual differences in brain activity make generalization difficult, meaning a model trained for one group of participants may not work well for another.
Nevertheless, the NYU study represents a stride in this direction by demonstrating high-accuracy speech decoding using lighterweight electrode arrays.
Looking ahead, the NYU team aims to refine their models for real-time speech decoding, bringing us closer to the ultimate goal of enabling natural, fluent conversations for individuals with speech impairments.
They also intend to adapt the system to implantable wireless devices that can be used in everyday life.