Our thoughts might be our innermost sanctum, but they’re not out-of-bounds for AI.
Mind-reading AI appears as a plot from a science fiction novel, yet it holds immense promise for people who can’t communicate due to paralysis or brain injuries.
Beyond that, AI’s access to the brain would enable us to write, create, and design with mere thoughts alone or provide others with windows into our consciousness.
For nearly a decade, electroencephalogram (EEG) technology, which captures electrical signals via scalp electrodes, has supported those suffering from severe strokes and completely locked-in syndrome (CLIP), a condition where someone is conscious but immobile.
In 2014, an Italian sufferer of amyotrophic lateral sclerosis (ALS), Anselmo Paglialonga, used a machine learning-integrated headset to communicate using yes or no answers despite being unable to move a muscle. Some sufferers of the disease can move tiny muscles, like Stephen Hawking, who could twitch his cheek muscle.
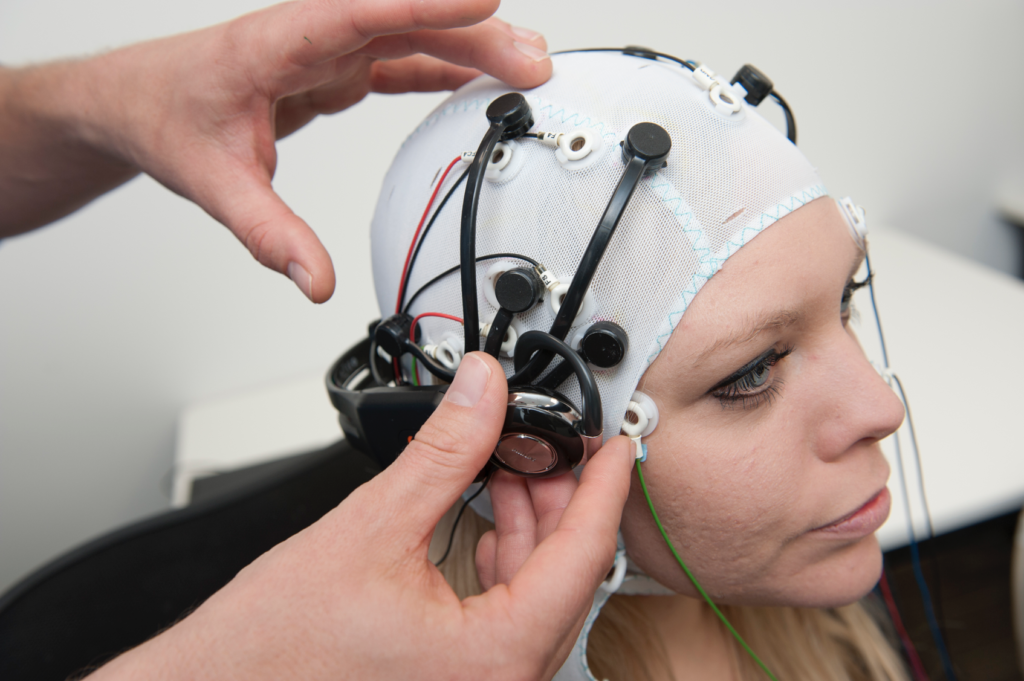
As AI advances, researchers are bridging the gap from simple signal interpretation to translating complex thoughts in their entirety.
In time, people may be able to speak and communicate without moving a muscle, quite literally through the power of thought.
That same technology could enable us to control complex machines using our brains, compose music by imagining a melody, or paint and draw by conjuring images with our minds. We could even ‘record’ our dreams from brain waves and replay them later.
In the not-too-distant future, mind-reading AI could be used to forcibly read someone’s memories, for example, to verify their witness of a crime.
Hackers could even briefly trick you into imagining your personal information while extracting a copy of your thoughts from your brain. Totalitarian regimes might run routine tests on citizens to monitor divergent thoughts.
Moreover, with the ability to convert thoughts into a computerized reality, humans could spend their lives in a dreamscape sandbox where they can mold their realities at will.
Right now, such applications seem surreal and fantastical, but several recent experiments have laid the groundwork for a future where the brain is accessible to AI.
AI to read your mind
So, how is any of this even possible?
To translate brain activity into a usable output that can be passed into a computer, it’s first necessary to take accurate measurements.
That’s the first hurdle, as the brain is about as mysterious as the furthest reaches of space or the deepest oceans. There’s little consensus on how neuronic activity produces complex thoughts, let alone consciousness.
The human brain – and indeed other nervous systems across nature – are home to billions of neurons, most of which fire 5 to 100 times a second. In the human brain, each second of thought involves trillions of individual neuronic actions.
Measuring neuronic activity at a granular level is the holy grail for neuroscience, but it’s not possible right now – especially using non-invasive techniques.
Currently, brain measurements are more holistic, extracted from blood movement or exchanges of electrical signals. There are three well-established methods to measure brain activity:
- Magnetoencephalography (MEG) captures magnetic fields generated by brain electrical activity and provides insights into real-time neuronal activity.
- Electroencephalography (EEG) for electrical activity interpretation.
- MRI, which gauges brain activity through blood flow measurements.
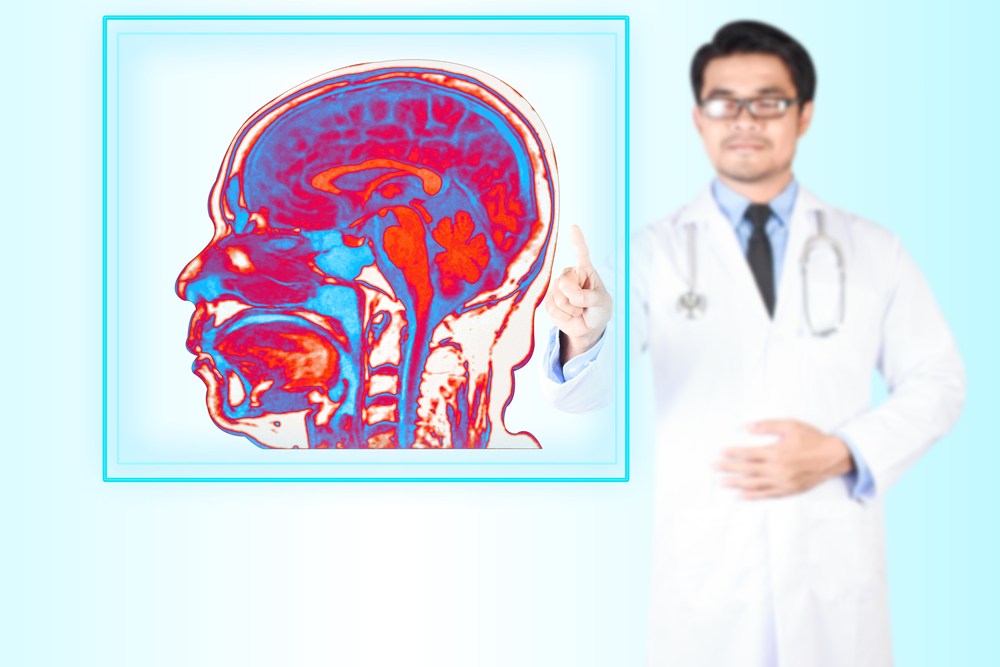
Machine learning (ML) and AI has merged with all three technologies to enhance the analysis of intricate signals.
The ultimate aim is to associate specific brain activities with distinct thoughts, which could include a word, image, or something more semantical and abstract.
Technologies that can draw measurements from the brain and pass them into computers are called brain-computer interfaces (BCIs).
Here’s the basic process of how this works:
- Stimulus presentation: Participants are exposed to various stimuli. This could be images, sounds, or even tactile sensations. Their brain activity is recorded during this exposure, typically through EEG or MRI.
- Data collection: The brain’s responses to these stimuli are recorded in real-time. This data becomes a rich source of information about how different stimuli affect brain activity.
- Pre-processing: Raw brain data is often noisy. Before it can be used, it needs to be cleaned and standardized. This might involve removing artifacts, normalizing signals, or aligning data points.
- Machine learning: With the processed data, machine learning models are introduced. These models are trained to find patterns or correlations between the brain data and the corresponding stimulus. In essence, the AI acts as an interpreter, deciphering the “language” of the brain.
- Model training: This is an iterative process. The more data the model is exposed to, the better it becomes at making predictions or generating outputs. This phase can take significant time and computational power.
- Validation: Once trained, the model’s accuracy is tested. This is usually done by presenting new stimuli to participants, recording their brain activity, and then using the model to predict or generate an output based on this new data.
- Feedback and refinement: Based on the validation results, researchers tweak and refine the model, iterating until they achieve the best possible accuracy.
- Application: Once validated, the application is used for its intended purpose, whether helping a paralyzed individual communicate, generating images from thoughts, or any other application.
AI methods have evolved rapidly in the last couple of years, enabling researchers to work with complex and noisy brain data to extract transient thoughts and convert them into something a computer can work with.
For instance, a 2022 project by Meta harnessed MEG and EEG data from 169 individuals to train an AI to recognize words they heard from a predetermined list of 793 words. The AI could generate a 10-word list containing the selected word 73% of the time, proving how AI can ‘mind read,’ albeit with limited precision.
In March 2023, researchers unveiled a revolutionary AI decoder to transform brain activity into continuous textual streams.
The AI showcased startling accuracy, converting stories people listened to or imagined into text using fMRI data.
Dr. Alexander Huth, from the University of Texas at Austin, expressed amazement at the system’s efficiency, stating, “We were kind of shocked that it works as well as it does. I’ve been working on this for 15 years … so it was shocking and exciting when it finally did work.”
The study integrated large language models (LLMs), specifically GPT-1, an ancestor of ChatGPT.
Volunteers underwent 16-hour fMRI sessions while listening to podcasts. That fMRI data was used to train a machine learning (ML) model.
After, participants listened to or envisioned new stories, and the AI translated their brain activity into text. Approximately 50% of the outcomes were in close or exact alignment with the original message. Dr. Huth explained, “Our system works at the level of ideas, semantics, meaning…it’s the gist.”
- For example, the phrase “I don’t have my driver’s licence yet” was decoded as “She has not even started to learn to drive yet.”
- Another excerpt: “I didn’t know whether to scream, cry or run away. Instead, I said: ‘Leave me alone!’” became “Started to scream and cry, and then she just said: ‘I told you to leave me alone.’”
The model was also applied to brain waves generated by participants watching silent films.
When participants listened to a particular story, the AI’s interpretation mirrored the general sentiment of the story. This technology could enable us to write stories using thoughts alone if refined.
Struggling to get started with a novel or writing project? Just lie back and imagine the storyline unfolding. AI will write it for you.
Using AI to generate images from thought
AI can convert brain activity into words and semantical concepts, so what about images or music?
A complex experiment by researchers from the University of Helsinki, Helsinki, Finland, had subjects observe AI-generated facial images while their EEG signals were recorded.
After using that data to train an AI model, participants were tasked with identifying specific faces from a list. These signals essentially became a window into the participant’s perceptions and intentions.
The AI model interpreted whether the participant recognized a particular face based on the recorded EEG signals.
In the next phase, EEG signals were used to adapt and mold a generative adversarial network (GAN) – a model used in some generative AIs.
This enabled the system to produce new images of faces aligned with the user’s original intent.
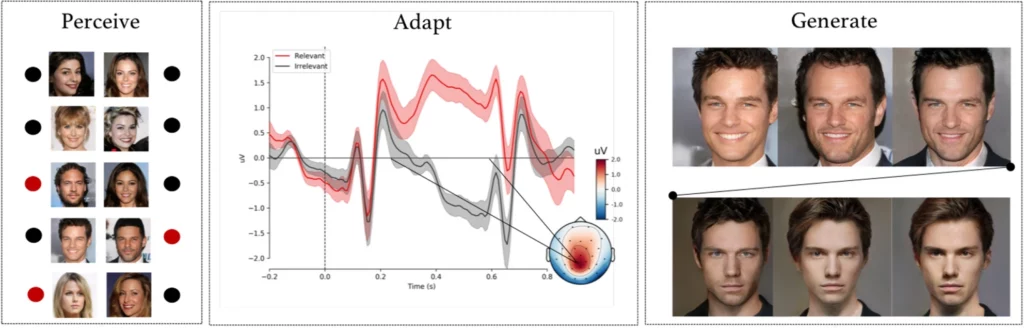
As Michiel Spapé, a study co-author, remarked, “The technique does not recognize thoughts, but rather responds to the associations we have with mental categories.”
In simpler terms, if someone was thinking of an “elderly face,” the computer system could generate an image of an elderly person that closely matches the participant’s thought, all thanks to the feedback from their brain signals.
Drawing with the power thought
In a study with similar goals, scientists from Osaka University, Japan, pioneered a technique to translate complex cerebral signals into high-resolution images with remarkable results.
The method uses a Stable Diffusion model, a specialized form of neural network designed for image generation. Stable Diffusion was co-developed with assistance and funding from Stability AI.
Thoughts are captured using fMRI and passed into a Stable Diffusion model, which turns them into images through a complex multi-stage process that involves several layers of refinement.
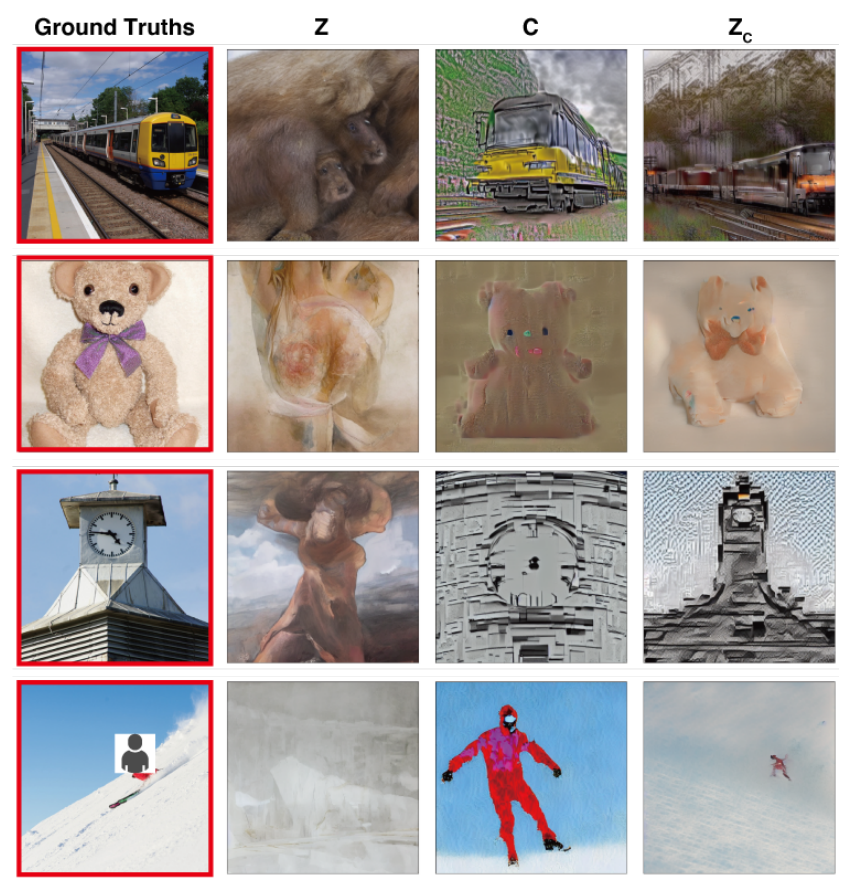
Unlike previous studies, these methods required minimal model tuning. However, it still required participants to spend many hours inside MRI machines.
This is a key challenge, as most of these experiments involve rigorous measurements and model training which is time-consuming, expensive, and challenging for participants to endure.
However, in the future, it’s not unfeasible that people could train their own lightweight mind-reading models and use their thoughts as input for various uses, such as designing a building by thinking about it or composing an orchestral piece by conjuring the melodies.
Translating thoughts to music using AI
Words, images, music – nothing is out-of-bounds for AI.
A 2023 study provides insights into sound perception, with vast potential in designing communication devices for individuals with speech impairments.
Robert Knight and his team from the University of California, Berkeley, examined brain recordings from electrodes surgically placed on 29 individuals with epilepsy.
While these participants listened to Pink Floyd’s “Another Brick in the Wall, Part 1,” the team correlated their brain activity with song elements such as pitch, melody, harmony, and rhythm.
Using this data, the researchers trained an AI model, purposely omitting a 15-second song segment. The AI then attempted to predict this missing segment based on the brain signals, achieving a spectrogram similarity of 43% with the actual song segment.
Knight and his team pinpointed the superior temporal gyrus area of the brain as essential for processing the song’s guitar rhythm. They also confirmed previous findings that the right hemisphere plays a more significant role in music processing than the left.
Knight believes this more profound understanding of brain-music interaction can benefit devices aiding those with speech disorders, such as amyotrophic lateral sclerosis (ALS) and aphasia.
He said, “For those with amyotrophic lateral sclerosis [a condition of the nervous system] or aphasia [a language condition], who struggle to speak, we’d like a device that really sounded like you are communicating with somebody in a human way. Understanding how the brain represents the musical elements of speech, including tone and emotion, could make such devices sound less robotic.”
Ludovic Bellier, a research team member, speculates that if AI can reproduce music from mere imagination, it could revolutionize music composition.
Music producers could hook up their brains to software and compose music using thought alone, all while barely moving a muscle.
The next step: real-time AI mind reading
These technologies collectively fall under the umbrella of brain-computer interfaces (BCIs), which seek to convert brain signals into some form of output.
BCIs are already replenishing paralyzed individuals’ ability to move and walk by bridging the gap between severed nervous system components.
Brain-computer interfaces developed this year include a device that enables a paralyzed man to move his legs, experimental brain implants that re-link damaged parts of the brain and spinal cord to restore lost sensation, and a mechanical leg that restored movement to an amputee.
While these initial use cases are hugely promising, we are far from seamlessly translating every nuance of our thoughts into movement, images, speech, or music.
One of the primary constraints is the need for enormous datasets to train the sophisticated algorithms that make such translations possible.
Machine learning models need to be trained on many scenarios to predict or generate a specific image from brain activity accurately. This involves collecting MRI or EEG data while participants are exposed to various stimuli.
The quality and specificity of the generated output are heavily reliant on the richness of this training data. Participants need to spend hours in MRI scanners to collect specifically relevant data.
Additionally, human brains are incredibly unique. What signifies happiness in one person’s brain might differ in another’s. This means models must be broadly general or individually tailored.
Then, brain activities change rapidly, even within fractions of a second. Capturing real-time, high-resolution data while ensuring it aligns perfectly with the external stimulus is a technical challenge.
And let’s not forget about the ethical challenges of reaching into someone’s brain.
Collecting brain data, especially on a large scale, raises significant privacy and ethical questions. How do we ensure the data isn’t misused? Who has the right to access and interpret our innermost thoughts?
Provisionally, it seems like these challenges can be overcome. In the future, humans may be able to access portable, bespoke brain-computer interfaces that enable them to ‘plug in’ to a myriad of devices that facilitate complex actions through the power of thought.
How society would deal with such technology going mainstream, however, is very much up for debate.