Apple is yet to officially release an AI model, but a new research paper gives an insight into the company’s progress in developing models with state-of-the-art multimodal capabilities.
The paper, titled “MM1: Methods, Analysis & Insights from Multimodal LLM Pre-training”, introduces Apple’s family of MLLMs called MM1.
MM1 displays impressive abilities in image captioning, visual question answering (VQA), and natural language inference. The researchers explain that careful choices of image-caption pairs enabled them to achieve superior results, especially in few-shot learning scenarios.
What sets MM1 apart from other MLLMs is its superior ability to follow instructions across multiple images and to reason on the complex scenes it’s presented with.
The MM1 models contain up to 30B parameters, which is three times that of GPT-4V, the component that gives OpenAI’s GPT-4 its vision capabilities.
Here are some examples of MM1’s VQA abilities.
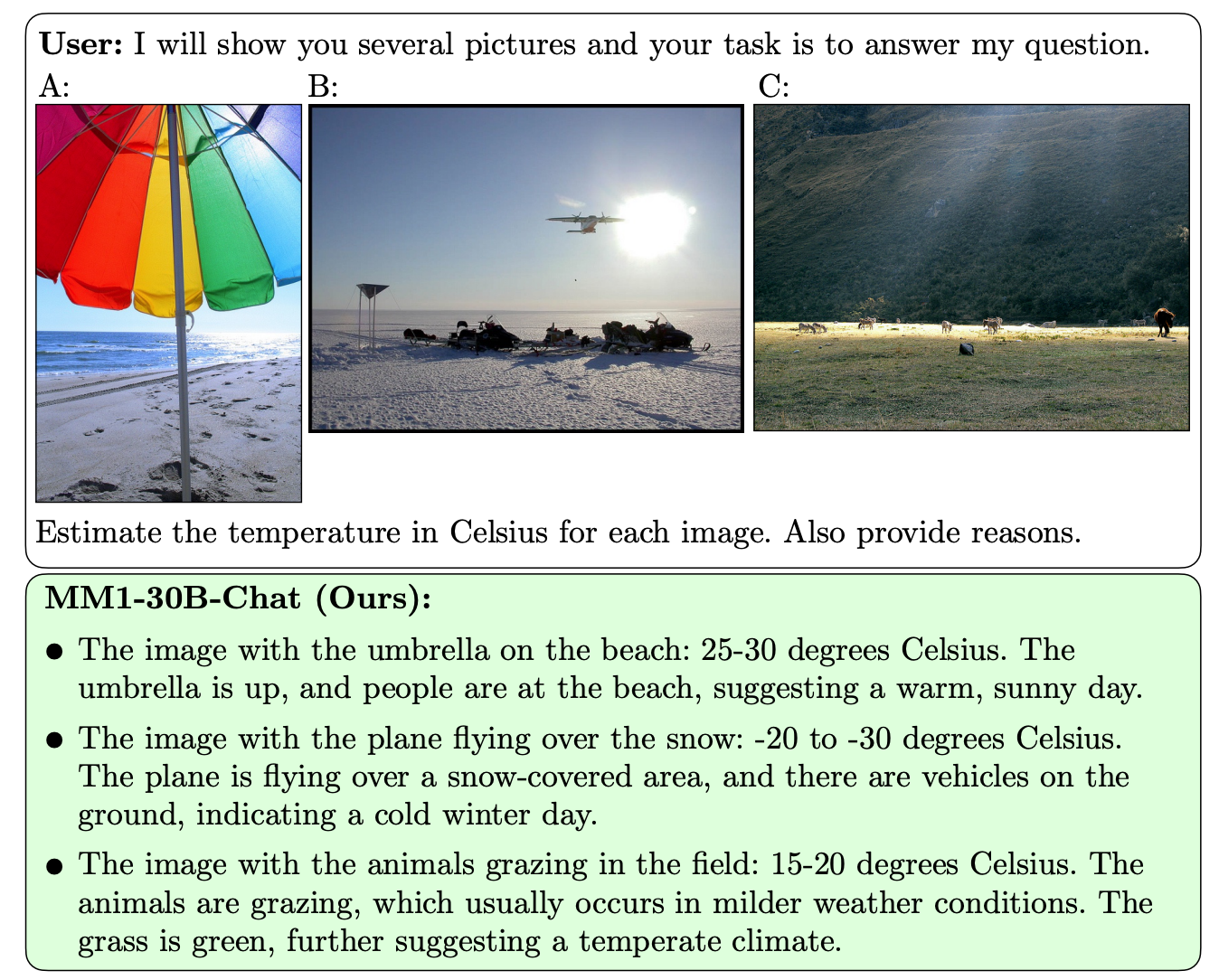
MM1 underwent large-scale multimodal pretraining on “a dataset of 500M interleaved image-text documents, containing 1B images and 500B text tokens.”
The scale and diversity of its pretraining enable MM1 to perform impressive in-context predictions and follow custom formatting with a small number of few-shot examples. Here are examples of how MM1 learns the desired output and format from just 3 examples.
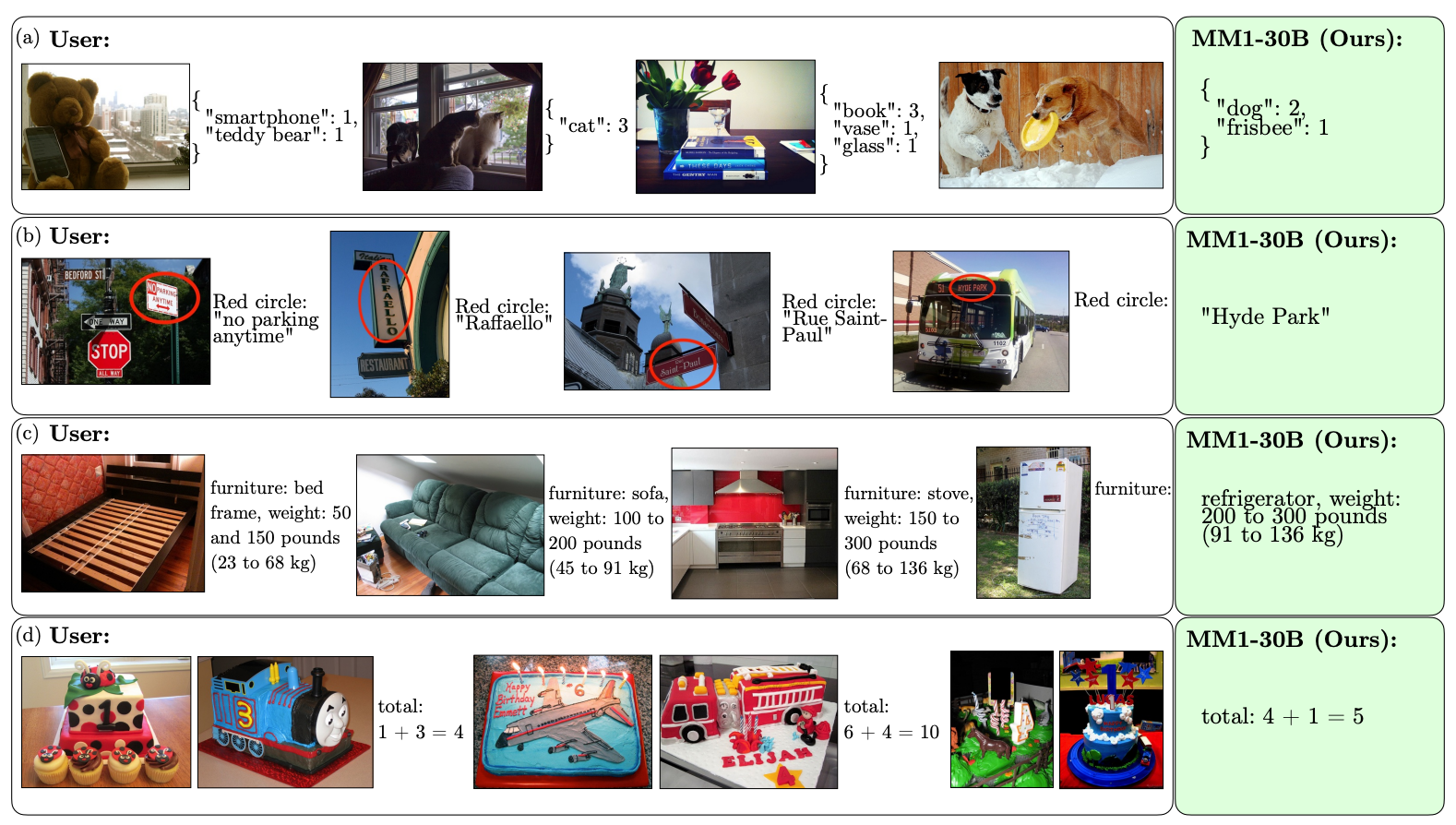
Making AI models that can “see” and reason requires a vision-language connector which translates images and language into a unified representation that the model can use for further processing.
The researchers found that the design of the vision-language connector was less of a factor in driving MM1’s performance. Interestingly, it was the image resolution and number of image tokens that had the biggest impact.
It’s interesting to see how open Apple has been in sharing its research with the broader AI community. The researchers state that “in this paper, we document the MLLM building process and attempt to formulate design lessons, that we hope are of use to the community.”
The published results will likely inform the direction other MMLM developers take regarding architecture and pre-training data choices.
Exactly how MM1 models will be implemented in Apple’s products remains to be seen. The published examples of MM1’s capabilities hint at Siri becoming a lot smarter when she eventually learns to see.