A study published in Reaction Chemistry and Engineering by researchers at the University of Surrey used AI to enhance carbon capture at power plants, reducing energy consumption by over a third.
The research, led by Professor Jin Xuan, chair of sustainable processes at the University of Surrey’s School of Chemistry and Chemical Engineering, focused on optimizing carbon capture systems.
It showcases a model system based on a real coal-fired power station that uses AI to achieve a 16.7% increase in carbon dioxide (CO2) capture while simultaneously reducing non-energy consumption from the UK’s national grid by 36.3%.
Prof. Xuan emphasized the novelty of this approach, stating in an article by the University of Surrey, “Usually, carbon capture systems run constantly, at the same rate—regardless of the externally changing environment. But we showed that teaching the system to keep making small adaptations can produce big energy savings—and capture more carbon at the same time.”
Carbon capture is a critical process in mitigating the environmental impact of CO2, the leading greenhouse gas produced by most power plants.
This is typically achieved through enhanced weathering, a method where flue gas is bubbled through water containing limestone, reacting with calcium carbonate to form harmless bicarbonate.
However, this process is energy-intensive, requiring power to pump water and CO2. The CO2 capture plant in the study had its own wind turbine for renewable energy, but in calmer weather where that was insufficient, it used energy from the grid.
The research team’s achievements lie in using AI to enable the model system to predict fluctuations in CO2 production and renewable energy availability. This allowed the system to adjust water pumping accordingly, optimizing energy use over time.
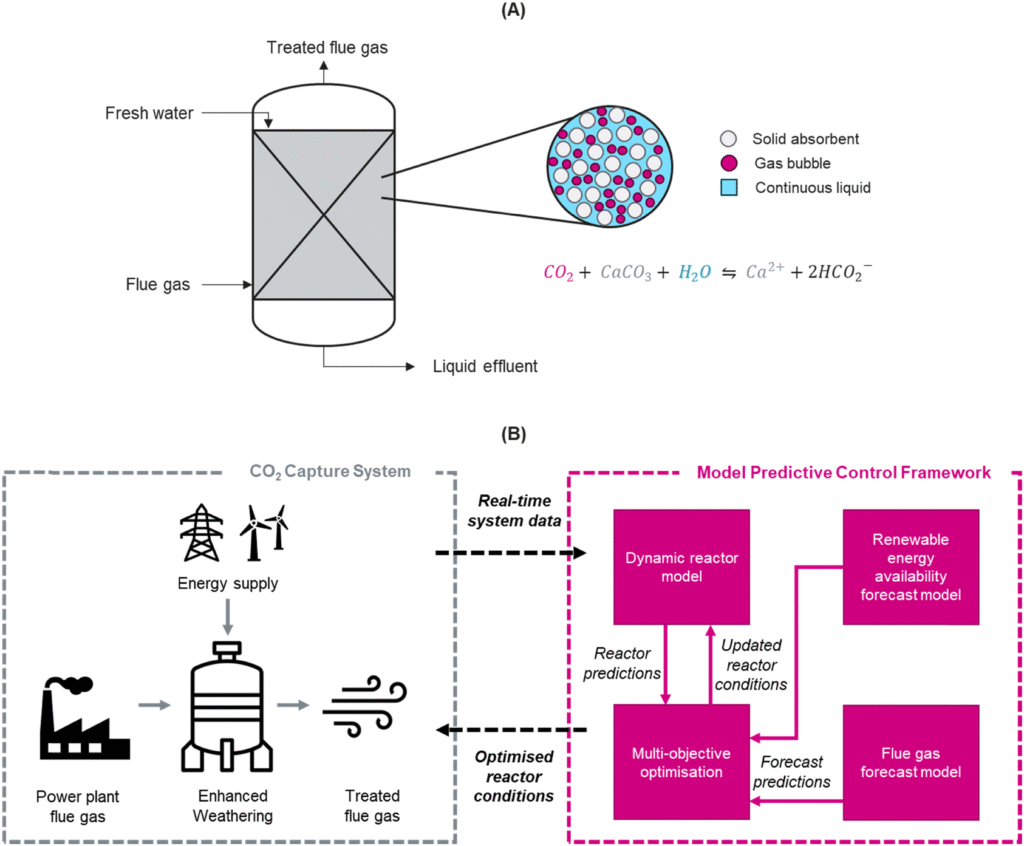
Dr. Lei Xing, a lecturer in chemistry and chemical engineering at the University of Surrey, highlighted the implications of their findings, saying, “Although we tested our model on enhanced weathering, the principles apply more widely. Our model could help anybody trying to capture and store more CO2 with less energy—whatever the process they’re using.”
The researchers hope these findings can contribute to the United Nations’ Sustainable Development Goals, which aim to reduce strain on non-renewable energy sources and invest in innovative carbon-capturing technologies.
Earlier in January this year, Microsoft used AI to create a new lithium battery electrolyte capable of reducing lithium content in batteries by some 70%.
More about the study
The study aimed to optimize carbon capture in order to reduce the use of non-renewable energy sources while maintaining or improving CO2 capture.
It used AI to adjust the system based on small changes rather than running continuously at the same rate.
Here’s how it works:
- Creating models for CO2 capture: Researchers developed machine learning models to predict how efficiently a reactor could capture CO2 and how much power it would use. They used two types of models: LSTM (Long Short-Term Memory) and MLP (Multilayer Perceptron).
- Forecasting key factors with AI: They used AI to predict two crucial factors: how much CO2 would be in the gas from a coal plant and how much wind energy would be available to power the capture process. These forecasts are important for planning the capture process.
- Testing and improving the models: The team rigorously tested their AI models for accuracy using statistical methods. They also used a technique called conformal prediction to determine how confident they could be in the model’s predictions.
- Optimizing the capture process: Using the data from their AI models, the researchers employed a sophisticated algorithm to find the best balance between capturing the most CO2 and using the least amount of non-renewable energy.
- Analyzing the results: The results were promising. They showed that, on average, the reactor captured 16.7% more CO2 and used 36.3% less energy from non-renewable sources over a month.
This demonstrates AI’s usefulness in energy reduction technology.
Lightweight AI systems fitted to energy-consuming processes and appliances can optimize their functionality to effectively lower resource consumption.