Nvidia scaled and optimized its Eos AI supercomputer to set new MLPerf AI training benchmark records.
When Nvidia switched its AI supercomputer Eos on in May, 10,752 NVIDIA H100 Tensor Core GPUs came to life and they’ve just been put through some real-world test trials.
This unprecedented processing power along with new software optimizations has enabled Eos to push the MLPerf benchmark into record territory.
The open-source MLPerf benchmark is a set of training and inference tests designed to measure the performance of machine learning workloads on real-world datasets.
One of the standout results was that Eos was able to train a GPT-3 model with 175 billion parameters on one billion tokens in just 3.9 minutes.
When Nvidia set the record on this benchmark less than 6 months ago it took almost 3 times longer with a time of 10.9 minutes.
Nvidia was also able to achieve a 93% efficiency rate during the tests, meaning it used almost all of the computing power theoretically available in Eos.
Microsoft Azure, which uses much the same H100 setup as Eos in its ND H100 v5 virtual machine, came within 2% of Nvidia’s test results in its MLPerf tests.
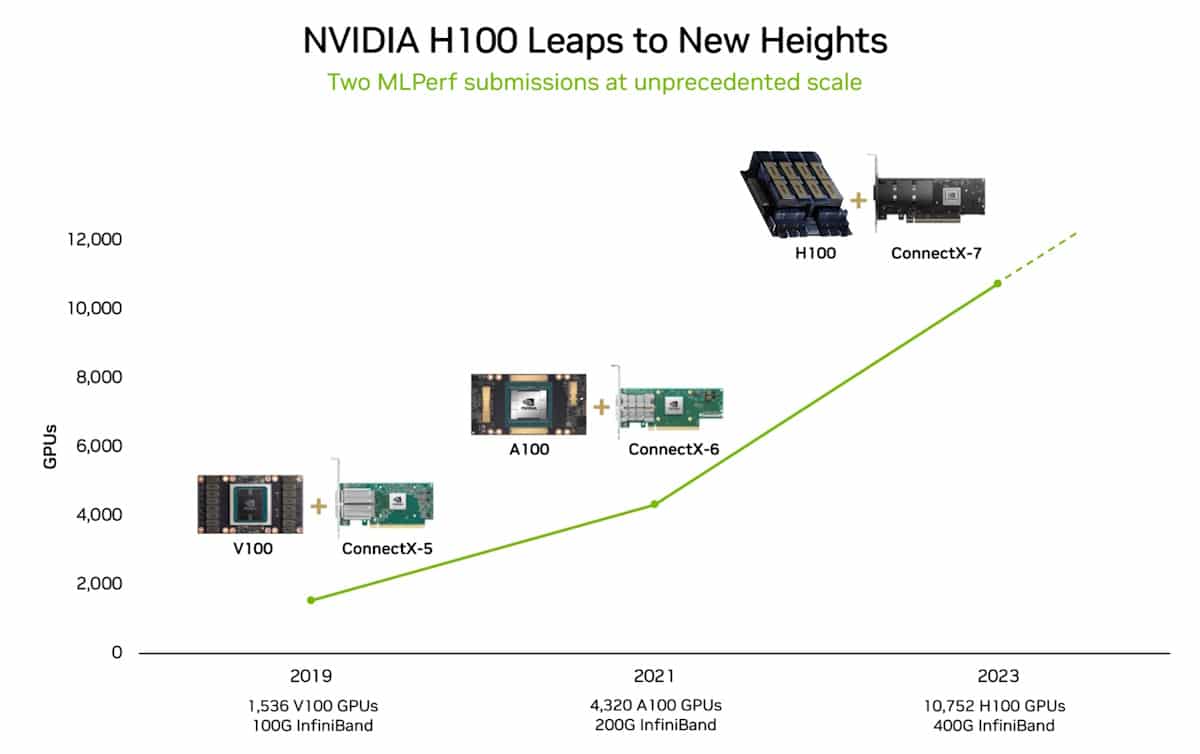
In 2018 Jensen Huang, the CEO of Nvidia, said that the performance of GPUs will more than double every two years. This claim was coined Haung’s Law and has proved true as it leaves Moore’s Law disappearing in the computing rearview mirror.
So what?
The MLPerf benchmark training test that Nvidia aced uses just a portion of the full dataset that GPT-3 was trained on. If you take the time Eos set in the MLPerf test and extrapolate for the full GPT-3 dataset then it could train the full model in just 8 days.
If you tried to do that using its previous state-of-the-art system made up of 512 A100 GPUs it would take around 170 days.
If you were training a new AI model, can you imagine the difference in time to market and cost that 8 days versus 170 days represents?
The H100 GPUs are not only a lot more powerful than the A100 GPUs, they’re up to 3.5 times more energy-efficient. Energy use and AI’s carbon footprint are real issues that need addressing.
To get an idea of how quickly AI processing is improving, think about ChatGPT which went live just under a year ago. The underlying model, GPT-3, was trained on 10,240 Nvidia V100 GPUs.
Less than a year later, Eos has 28 times the processing power of that setup with a 3.5x improvement in efficiency.
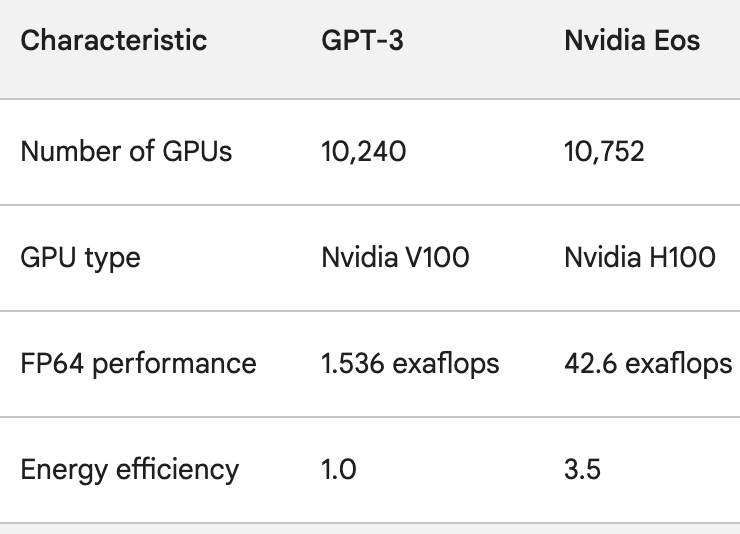
When OpenAI’s Sam Altman concluded the recent DevDay he said that the projects OpenAI was working on would make its latest releases look quaint.
Considering the leap in processing power companies like Nvidia are achieving, Altman’s claim likely sums up the future of the AI industry as a whole.