The Amazon Rainforest, covering a vast 2.3 million square miles, is the world’s largest rainforest and most biodiverse region.
Spread across nine countries, the Amazon is essential for the planet’s health, absorbing carbon dioxide, regulating weather patterns, and providing a habitat for innumerable species.
Deforestation and illegal land use have threatened the Amazon for decades, leading to large-scale habitat loss and near-irreversible ecosystem damage.
According to Amazon Conservation, nearly 5 million acres of rainforest were lost in 2022 alone, marking a 21% increase from the previous year.
AI solutions for conservation
Deep in the Amazon rainforest, animals rummage through the undergrowth without realizing they’re being captured by cameras and microphones.
The images and recordings don’t just provide a tantalizing insight into the lives of animals in the rainforest – they form part of a sophisticated AI-driven project to solve deforestation.
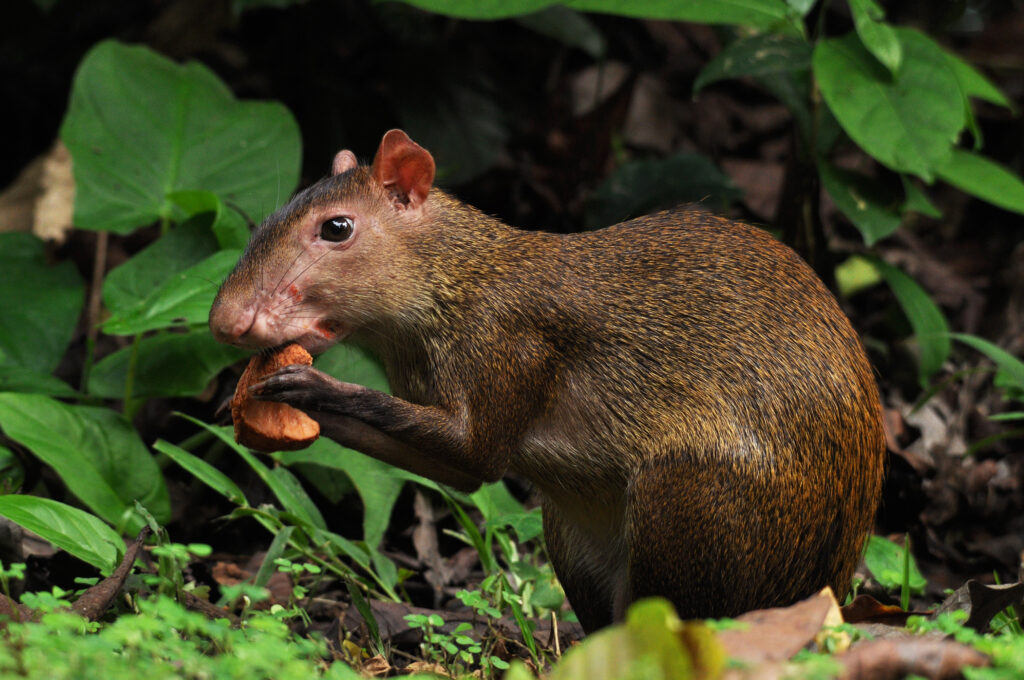
Leveraging the power of data, machine learning (ML), and cloud technology, experts are developing innovative programs aimed at recognizing deforestation patterns and providing actionable data for policymakers.
AI has become integral to global conservation efforts, providing researchers with a means of combining data from sensors, cameras, and satellites.
Project Guacamaya, a collaboration between Alexander von Humboldt Institute, the CinfonIA Research Center at Universidad de los Andes, Instituto Sinchi, and Microsoft’s AI for Good Lab, aims to monitor deforestation and biodiversity in the Colombian Amazon.
As Juan Lavista Ferres, Vice President and Chief Data Scientist at Microsoft’s AI for Good Lab, describes, “This project is not going to solve all the problems the Amazon has, but it is going to solve one I think is fundamental: You can’t solve a problem if you can’t measure it.”
A three-pronged approach
AI’s ability to work with data across multiple modalities equips researchers with a detailed account of this vast and complex environment.
For instance, satellite data provides a macro analysis of the forest, including deforestation events, illegal mining, and changes in land use.
Simultaneously, cameras and sensors on the ground track macro changes’ impacts on local biodiversity, such as tracking habitat loss.
Here’s how Project Guacamaya combines different AI systems:
Step 1: Satellite data for macro land analysis
The first component of Project Guacamaya is leveraging satellite data from Planet Labs.
Satellite data supplies the project with daily high-resolution images of the Amazon Rainforest, enabling near-real-time monitoring. This is crucial for detecting rapid changes in forest cover or evidence of illegal activities.
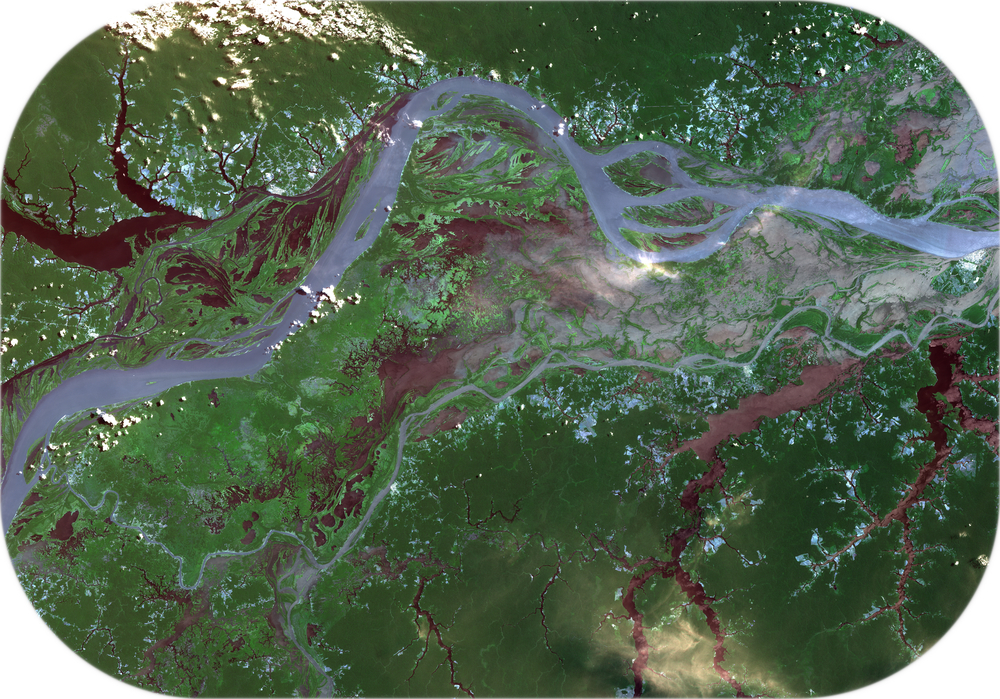
The AI models developed for this phase are trained to search for deforestation or illegal mining indicators, such as unauthorized roads or clearings.
By automating satellite surveillance, the team can alert Colombian authorities almost as soon as any suspicious activity begins.
Step 2: Hidden cameras for ground-level insight
Hidden cameras are strategically placed throughout the Colombian Amazon to supplement satellite data.
These cameras capture thousands of photos daily and feed them into AI models that identify and classify animals.
In addition to tracking species movement on the rainforest floor, this also serves as an alert system. For example, if animals are found outside their natural ecosystems, this could indicate local changes requiring further investigation.
Step 3: Bioacoustics for animal classification
Finally, Project Guacamaya incorporates sound data, or bioacoustics, captured directly from the Amazon Rainforest.
Specialized equipment is used to record the natural sounds of the forest, feeding data into AI models trained to distinguish between bird and non-bird sounds and classify them into specific species.
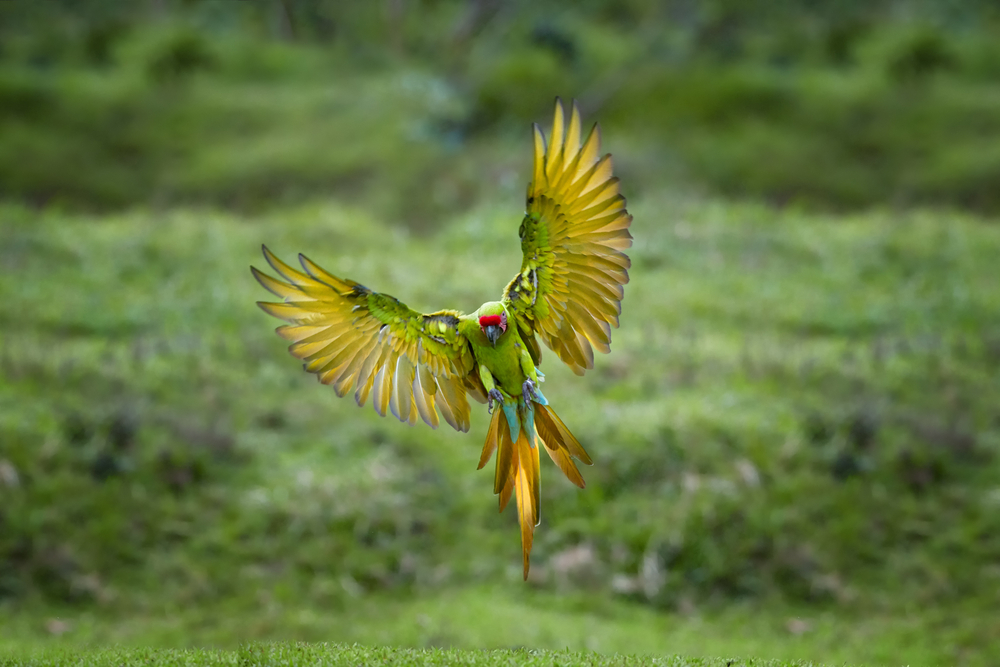
With identification reliability of over 80%, these AI models help scientists understand animal behaviors, track species migration, and detect the presence of invasive or endangered species.
Tackling deforestation in Brazil
In a separate project, Microsoft is collaborating with environmental organization Imazon and nonprofit PrevisIA in Brazil to detect illegal mining and deforestation via satellite analysis.
“We use PrevisIA to anticipate the risk areas and implement actions to avoid deforestation,” said Carlos Souza, a senior researcher with Imazon.
Earlier this year, the forest region in Triunfo do Xingu was decimated, losing an area equivalent to 700 football fields in just one month. According to PrevisIA, it’s also the region at the highest risk for further deforestation in 2023.
By the end of the year, the AI estimates that approximately 271.52 square kilometers of forest will be lost.
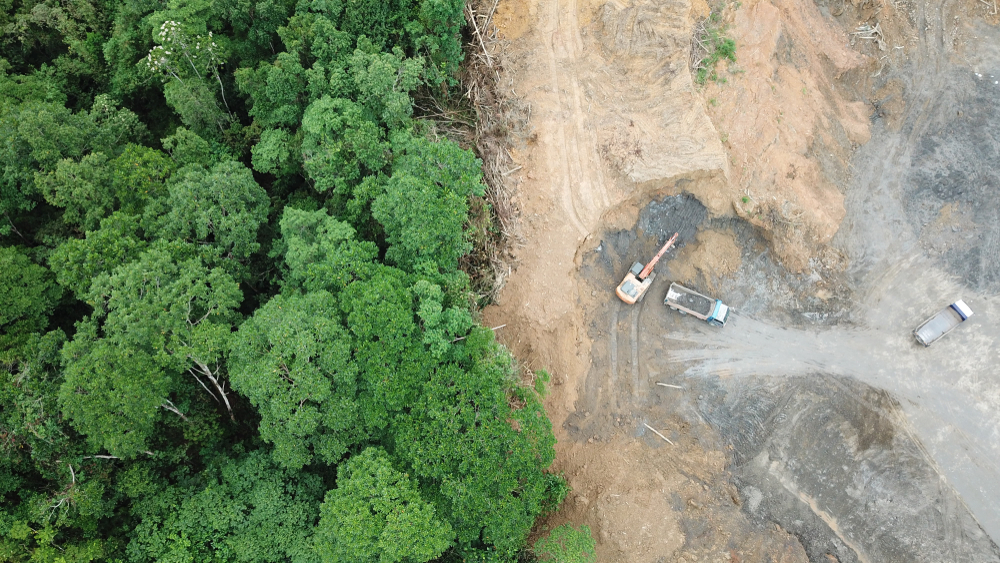
Carlos Souza Jr., senior researcher at Imazon and project coordinator of PrevisIA, highlighted the proactive power of this approach: “Existing deforestation prediction models were long-term, looking at what would happen in decades. We needed a new tool that could get ahead of the devastation.”
Using a combination of geostatistics and historical data, the model considers variables that inhibit or promote deforestation, such as land protected by indigenous communities.
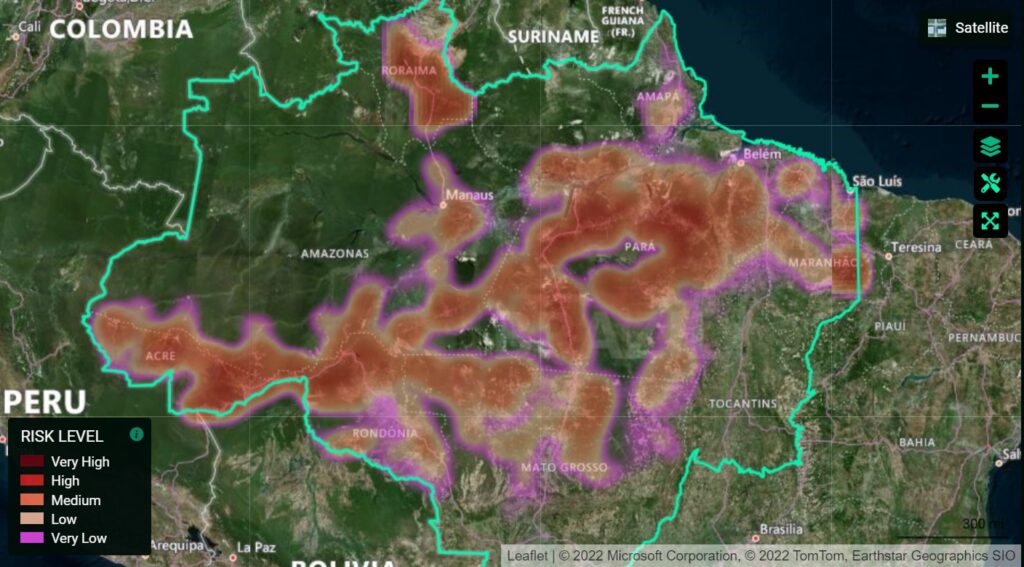
PrevisIA also benefits local stakeholders, including banks and businesses, who use the data to make environmentally responsible decisions.
From reactive to proactive conservation
AI’s ability to work with complex data in near-real-time supports a new paradigm of proactive conservation.
Prior, researchers would rely primarily on manually collected field data, which can’t hope to capture the dynamics of an area as vast as the Amazon.
José Godofredo Pires dos Santos, a public prosecutor in Pará, described the need for preventive measures, stating, “We don’t want to have to keep coming in after the damage has already been done.”
Researchers note that the ultimate goal is to make these models open-source for use in other global projects.
AI’s role in conservation: examples from 2023
In a world grappling with climate change, habitat loss, and biodiversity decline, traditional conservation methods often fall short.
2023 has seen a variety of fascinating conservation projects that harness AI and machine learning.
Here are three examples from the past few months:
Acoustic monitoring of Amazon river dolphins
Researchers at the Technical University of Catalonia in Barcelona trained a neural network to differentiate between two endangered species of dolphins in the Amazon River – Boto and Tucuxi – based on their unique acoustic communications.
By installing underwater microphones across the Mamirauá reserve in the Brazilian Amazon Rainforest, the team can monitor river dolphins with minimal disruption.
The AI technology helps distinguish dolphin sounds from other environmental noises.
Norway’s AI-powered fish immigration control
Pacific salmon, an invasive species, threatens native Atlantic salmon populations in European waterways.
Huawei and Berlevåg Jeger-og Fiskerforening (BJFF) built an AI fish filtering system in Norway’s Storelva River.
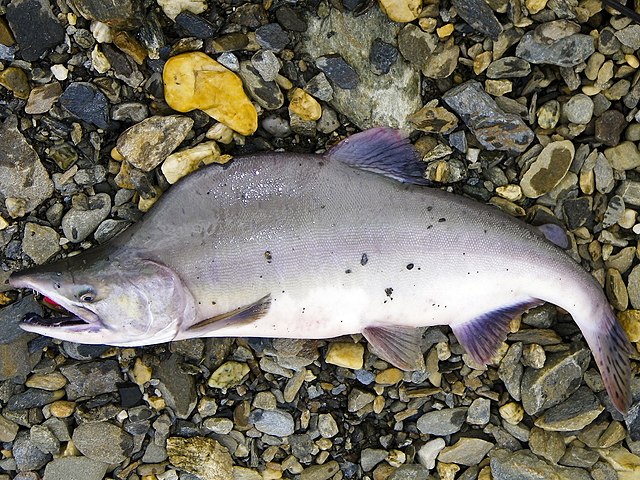
Using a computer vision (CV) model, this system can differentiate between Atlantic and Pacific salmon with 90% accuracy, diverting the invasive species into a holding bay before releasing them at sea.
This innovative system ‘sees’ the invasive Pacific salmon and safely prevents them from invading European waterways.
Counting Puffins in the UK
Puffins, beloved but vulnerable seabirds in the UK, have historically been challenging to monitor.
Rangers traditionally counted them manually – an arduous, time-consuming task.
In partnership with Microsoft, Avanade, and NatureScot, SSE Renewables is piloting an AI system to count puffins on the Isle of May, off the coast of Scotland.
Cameras capture live footage of puffins, and an AI model trained on labeled images can distinguish individual birds with minimal human interference.
The future of AI in conservation
The burgeoning role of AI in conservation has proven its effectiveness across varied ecosystems – from the Amazon River to European waterways and Scottish coastlines.
AI is a force multiplier, helping researchers stay on top of complex biodynamic data.
As the infrastructure required to train and deploy AI models becomes more accessible, building models for unique conservation problems will become easier, even without significant resources.
In the future, conservationists will be able to build sophisticated, lightweight AI systems with minimal expertise.