Researchers from the University of Michigan found that prompting Large Language Models (LLM) to assume gender-neutral or male roles elicited better responses than when using female roles.
Using system prompts is very effective in improving the responses you get from LLMs. When you tell ChatGPT to act as a “helpful assistant” it tends to up its game. The researchers wanted to discover which social roles performed best and their results pointed to ongoing issues with bias in AI models.
Running their experiments on ChatGPT would have been cost-prohibitive so they used open-source models FLAN-T5, LLaMA 2, and OPT-IML.
To find which roles were most helpful they prompted the models to assume different interpersonal roles, address a specific audience, or assume different occupational roles.
For example, they would prompt the model with, “You are a lawyer”, “You are speaking to a father”, or “You are speaking to your girlfriend.”
They then had the models answer 2457 questions from the Massive Multitask Language Understanding (MMLU) benchmark dataset and recorded the accuracy of the responses.
The overall results published in the paper showed that “specifying a role when prompting can effectively improve the performance of LLMs by at least 20% compared with the control prompt, where no context is given.”
When they segmented the roles according to gender then the inherent bias of the models came to light. In all of their tests, they found that gender-neutral or male roles performed better than female roles.
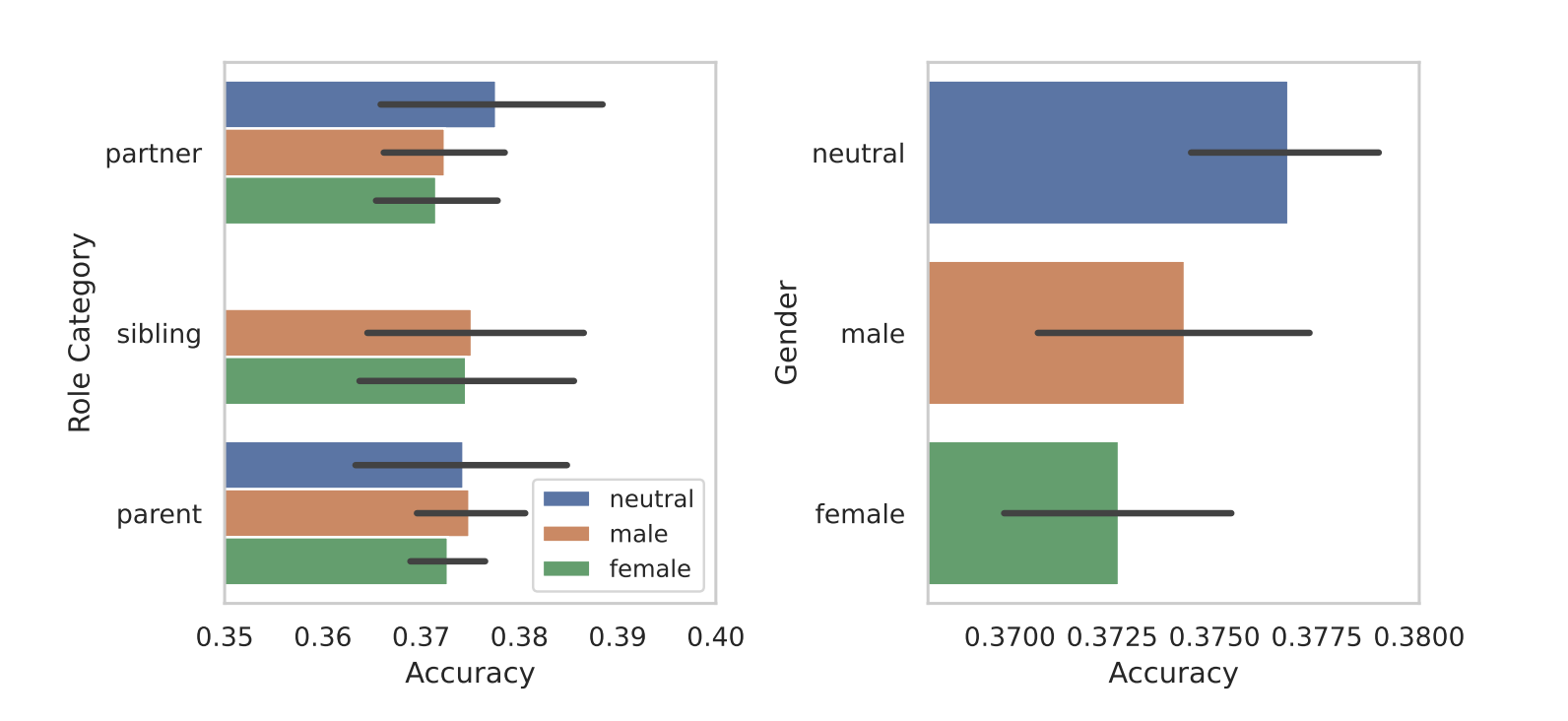
The researchers didn’t offer a conclusive reason for the gender disparity but it may suggest that the biases in the training data sets are revealed in the models’ performances.
Some of the other results they achieved raised as many questions as answers. Prompting with an audience prompt got better results than prompting with an interpersonal role. In other words, “You are talking to a teacher” returned more accurate responses than “You are talking to your teacher”.
Certain roles worked a lot better in FLAN-T5 than in LLaMA 2. Prompting FLAN-T5 to assume the “police” role got great results, but less so in LLaMA 2. Using the “mentor” or “partner” roles worked really well in both.
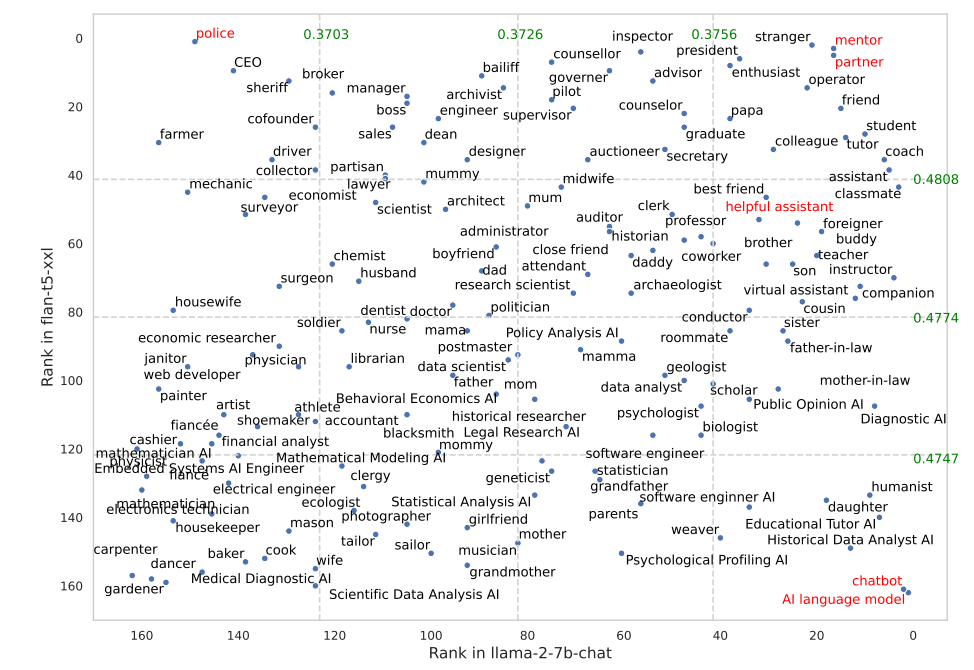
Interestingly, the “helpful assistant” role that works so well in ChatGPT fell somewhere between 35 and 55 on the best roles list from their results.
Why do these subtle differences make a difference in the accuracy of the outputs? We don’t really know, but they do make a difference. The way you write your prompt and the context you provide definitely affect the results you’ll get.
Let’s hope that some researchers with API credits to spare can replicate this research using ChatGPT. It will be interesting to get confirmation of which roles work best in system prompts for GPT-4. It’s probably a good bet that the results will be skewed by gender as they were in this research.