Researchers have introduced FANToM, a novel benchmark designed to rigorously test and evaluate large language models’ (LLMs) understanding and application of Theory of Mind (ToM).
Theory of Mind refers to the ability to attribute beliefs, desires, and knowledge to oneself and others, and understanding that others have beliefs and perspectives different from one’s own.
ToM is viewed as foundational to the consciousness possessed by intelligent animals. In addition to humans, primates such as orangutans, gorillas, and chimpanzees are considered to have ToM, as well as some non-primates such as parrots and members of the corvid (crow) family.
As AI models become more complex, AI researchers are seeking new methods of evaluating abilities such as ToM.
A new benchmark called FANToM, created by researchers from the Allen Institute for AI, the University of Washington, Carnegie Mellon University, and Seoul National University, subjects machine learning models to dynamic scenarios reflective of real-life interactions.
With FANToM, characters enter and exit conversations, challenging AI models to maintain an accurate understanding of who knows what at any given moment.
Subjecting large language models (LLMs) to FANToM revealed that even the most advanced models struggle with maintaining a consistent ToM.
The models’ performance was significantly lower than that of human participants, highlighting the limitations of AI in understanding and navigating complex social interactions.
In fact, humans dominated every category, as viewed below.
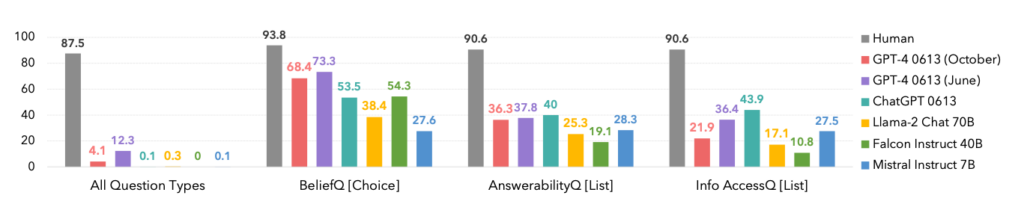
An interesting side point is that the October version of the GPT-4 model iteration was outperformed by an earlier June, which could back up recent anecdotes among users that ChatGPT is getting worse.
FANToM also revealed techniques for improving LLM ToM, such as chain-of-thought reasoning and other fine-tuning methods.
However, the gap between AI and human ToM skills remains high.
AI leaps toward human-like language skills
In a somewhat related but separate study published in Nature, scientists developed a neural network capable of human-like language generalization.
This new neural network showcased an impressive ability to integrate newly learned words into its existing vocabulary. It could then use those words in various contexts, a cognitive skill known as systematic generalization.
Humans naturally exhibit systematic generalization, seamlessly incorporating new vocabulary into their repertoire.
For example, once someone learns the term ‘photobomb,’ they can apply it in various situations almost immediately. New slang pops up all the time, and humans naturally absorb it into their vocabulary.
The researchers subjected both their own custom neural network and ChatGPT to a series of tests, finding that ChatGPT lagged behind the custom model in performance.
While LLMs like ChatGPT excel in many conversational scenarios, they exhibit noticeable inconsistencies and gaps in others, an issue this new neural network addresses.
To investigate this aspect of linguistic communication, researchers conducted an experiment involving 25 human participants, assessing their ability to apply newly learned words in different contexts. The subjects were introduced to a pseudo-language consisting of nonsense words representing various actions and rules.
After a training phase, the participants excelled in applying these abstract rules to new situations, showcasing systematic generalization.
When the newly developed neural network was exposed to this task, it mirrored human performance. However, when ChatGPT was subjected to the same challenge, it struggled significantly, failing between 42 and 86% of the time, depending on the specific task.
This is significant for two reasons. Firstly, you could argue this new neural network effectively outperformed GPT-4 on this specific task – which is impressive enough. Secondly, this study exposes new methods to teach AI models how to generalize new language like humans.
As Elia Bruni, a specialist in natural language processing at the University of Osnabrück in Germany, describes, “Infusing systematicity into neural networks is a big deal.”
Together, these two studies offer new approaches to training more intelligent AI models that can rival humans in critical areas such as linguistics and Theory of Mind.