Researchers from UK universities Durham University, University of Surrey, and Royal Holloway University of London developed an innovative AI-supported technique for extracting keystrokes from acoustic recordings.
The study developed a technique to successfully read keystrokes from keyboards using audio recordings.
This technique could enable hackers to analyze keystrokes by taking over a device’s microphone and collecting personal information such as passwords, private conversations, messages, and other sensitive data.
Keystrokes are recorded through a microphone and processed and analyzed by a machine learning (ML) model that determines their spacing and positionality on the keyboard.
The model can identify individual keystrokes with a shocking 95% accuracy rate when strokes are recorded through a nearby phone microphone. The prediction accuracy decreased to 93% when recordings made through Zoom were used to train the sound classification algorithm.
Acoustic hacking attacks have become increasingly sophisticated due to the widespread availability of devices equipped with microphones that can capture high-quality audio.
How does the model work?
The attack begins by recording keystrokes on the target’s keyboard. This data is crucial for training the predictive algorithm.
This recording can be accomplished using a nearby microphone or the target’s malware-infected phone with access to its microphone.
Alternatively, a rogue participant in a Zoom call could correlate messages typed by the target with their sound recording. There may also be ways of hacking the computer’s microphone using malware or software vulnerabilities.
The researchers gathered training data by pressing each of the 36 keys on a modern MacBook Pro 25 times, recording the sound each keypress generated. Waveforms and spectrograms were produced from these recordings, visualizing identifiable variations for each key.
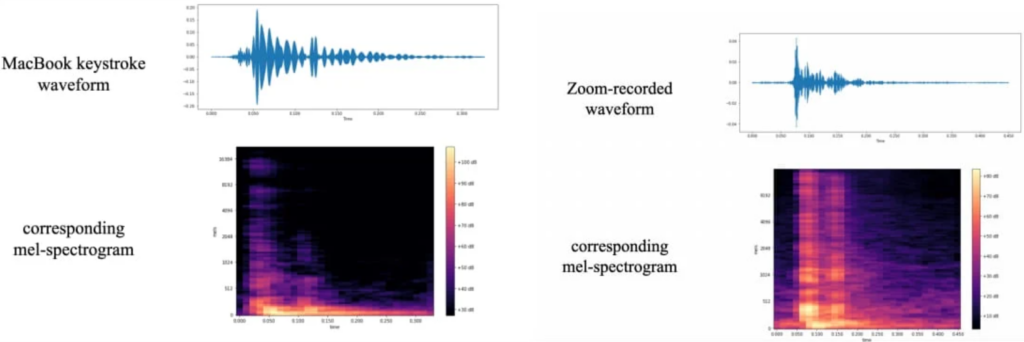
Further data processing was performed to enhance the signals used for identifying keystrokes.
These spectrogram images were used to train ‘CoAtNet,’ an image classifier that attributes different audio spectrograms to different keystrokes.
In their tests, the researchers used a laptop with a keyboard similar to newer Apple laptops. Microphones and recording methods included an iPhone 13 Mini placed 17 cm away from the target, Zoom, and Skype.
The CoANet classifier demonstrated 95% accuracy from smartphone recordings and 93% from those captured via Zoom. They also tested keystrokes recorded through Skype, which yielded 91.7% accuracy.
The research paper suggests altering typing styles or using randomized passwords to prevent such attacks, but that’s far from practical.
Other potential defensive measures include employing software to reproduce keystroke sounds, white noise, or software-based keystroke audio filters. However, the study found that even a silent keyboard could be successfully analyzed for keystrokes.
This is another novel example of how machine learning can enable sophisticated fraud techniques. A recent study found that deep fake audio voices could fool as many as 25% of people.
Audio attacks could be targeted at high-profile individuals such as politicians and CEOs to steal sensitive information or launch ransomware attacks based on thieved conversations.