Researchers at Mount Sinai have used machine learning to develop a novel electrocardiogram (ECG) analysis model called HeartBEiT.
HeartBEiT was pre-trained on a colossal dataset of 8.5 million ECGs from 2.1 million patients and outperformed classic convolutional neural networks (CNNs).
ECGs are ubiquitous heart diagnostic tests, and some 100 million are performed each year in the US alone.
An ECG measures electrical activity from the heart and is highly informative of a huge range of rhythm disorders, abnormal heart activity associated with heart attacks, and other forms of heart disease. The issue with ECGs is many of the subtler indications are tough to interpret, especially for rare heart conditions.
Prior to this study, machine learning (ML) had already been applied to ECG images to automatically flag and classify abnormal results. This increases diagnostic speed and accuracy and reduces the chance of clinical oversight.
Mount Sinai, a New York hospital and research network, trained a vision-based transformer model on 8.5 million ECG scans.
Notably, the model differs from convolutional neural networks (CNNs), the most common neural network for image classification tasks. The study, published in Nature, states that the model outperformed other models for identifying various heart conditions.
How HeartBEiT works
CNNs for image classification involve supervised machine learning, meaning they require large quantities of labeled data, which can be labor-intensive to collect and annotate. Conversely, HeartBEiT uses unsupervised techniques to learn from a vast dataset, providing a foundation for downstream tuning to more specific tasks.
The research team used the DALL-E model, created by OpenAI. DALL-E learns the relationships between tokens. In this case, parts of the ECG images – the tokens – are broadly analogous to words in a sentence.
The model learns their relationship to each other, which allows it to single out abnormal tokens that indicate cardiac problems.
HeartBEiT’s predictions were compared against standard CNN architectures for several heart conditions, outperforming them over smaller sample sizes.
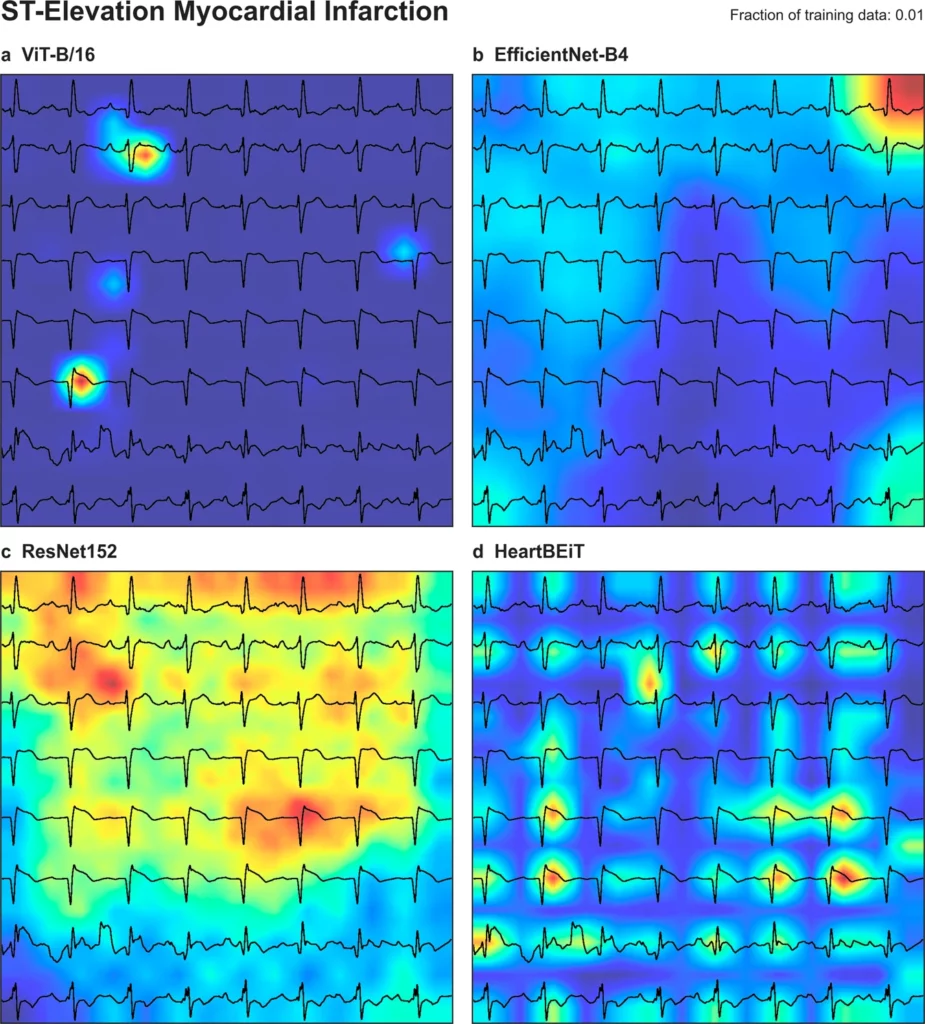
One of the authors, Akhil Vaid, Instructor of Data-Driven and Digital Medicine (D3M) at the Icahn School of Medicine at Mount Sinai, said: “Our model consistently outperformed convolutional neural networks [CNNs], which are commonly used machine learning algorithms for computer vision tasks.”
Additionally, HeartBEiT was able to highlight the specific region of the ECG associated with the abnormality.
Another author, Girish Nadkarni, MD, MPH, Director of The Charles Bronfman Institute of Personalized Medicine, said, “Neural networks are considered black boxes, but our model was much more specific in highlighting the region of the ECG responsible for a diagnosis, such as a heart attack, which helps clinicians to better understand the underlying pathology.”
“By comparison, the CNN explanations were vague even when they correctly identified a diagnosis.”
AI’s role in medical research and development is well-established, and this is another example of the innovative repurposing of machine learning (ML) models for medical applications.