Researchers at the University of Cambridge have harnessed AI to dramatically accelerate the search for new Parkinson’s disease therapies.
By using machine learning techniques, they were able to screen millions of potential drug compounds and identify the most promising candidates ten times faster and 1000 times more cost-effectively than conventional methods.
Parkinson’s disease is a complex, progressive neurodegenerative disease that afflicts approximately 6 million people worldwide. That figure is expected to triple by 2040.
Currently, no treatments can reliably slow or halt the disease’s progression.
The traditional process of screening vast chemical libraries to find potential drug candidates is extremely slow, expensive, and often unsuccessful.
“One route to search for potential treatments for Parkinson’s requires the identification of small molecules that can inhibit the aggregation of alpha-synuclein, which is a protein closely associated with the disease,” lead researcher Professor Michele Vendruscolo told the University of Cambridge.
“But this is an extremely time-consuming process – just identifying a lead candidate for further testing can take months or even years.”
To tackle this challenge, Vendruscolo and his team developed a 5-step machine learning approach. The study was published in Nature Chemical Biology.
- Start with a small set of compounds, identified via simulations, that show potential to block clumping of the alpha-synuclein protein, which is the primary cause of Parkinson’s. Then, experimentally test their effectiveness.
- Use the results to train a machine learning model to predict what molecular structures and properties make a compound effective at preventing protein aggregation.
- Deploy the trained model to rapidly screen a virtual library containing millions of compounds and predict the most potent contenders.
- Experimentally validate the top AI-selected candidates in the lab. Feed these results back into the model to refine its prediction capabilities further.
- Repeat this cycle of computational prediction and experimental testing, with the AI model getting smarter each round, zeroing in on the most powerful compounds.
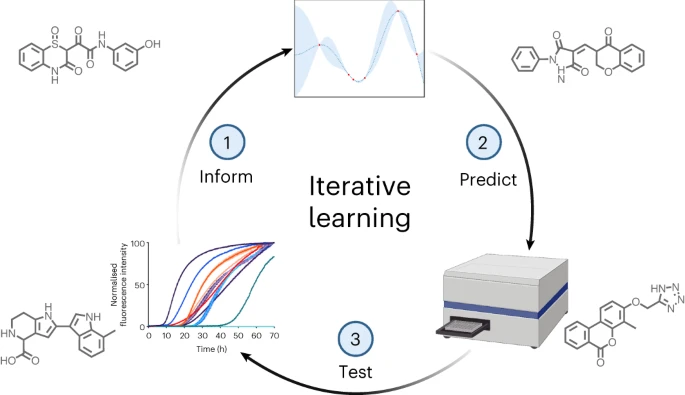
Over multiple iterations, the optimization rate – the percentage of tested compounds that inhibited alpha-synuclein clumping associated with Parkinson’s – increased from 4% to over 20%.
What’s more, the compounds found by the AI were, on average, far more potent than any previously identified. Some showed promising activity at eight-fold lower doses. They were also more chemically diverse, with the model discovering effective compounds that differed from known structures.
“Machine learning is having a real impact on drug discovery – it’s speeding up the whole process of identifying the most promising candidates,” said Vendruscolo.
“By using the knowledge we gained from the initial screening with our machine learning model, we were able to train the model to identify the specific regions on these small molecules responsible for binding, then we can re-screen and find more potent molecules.”
“For us, this means we can start work on multiple drug discovery programs – instead of just one. So much is possible due to the massive reduction in both time and cost – it’s an exciting time.”
The researchers emphasize this is just the beginning of what AI-first approaches could enable in drug discovery for Parkinson’s and other diseases characterized by protein misfolding and aggregation.
With further development and larger training datasets, the predictive power of these models should only improve.
While there’s still a long road ahead to turn these AI-identified candidates into approved treatments, this study demonstrates how machine learning, cleverly combined with experimental biology, can greatly accelerate the early stages of drug discovery.
This builds on a raft of research tackling the challenge of locating new, novel drug treatments, including from MIT and Tufts, that recently built a model capable of sifting through some 100 million compounds daily.
Several antibiotic discovery models have produced experimental compounds, some of which are heading to clinical trials.
Another large-scale project in collaboration with the Moorfields Eye Hospital in the UK from last year used eye scans to identify the early signs of Parkinson’s – a novel method enabled by AI.
With this new study that aims to discover effective Parkinson’s treatments, AI methods show immense promise in redefining medicine and healthcare.